Learning Generalizable Visual Representations via Interactive Gameplay
Authors
Abstract
A growing body of research suggests that embodied gameplay, prevalent not just in human cultures but across a variety of animal species including turtles and ravens, is critical in developing the neural flexibility for creative problem solving, decision making, and socialization. Comparatively little is known regarding the impact of embodied gameplay upon artificial agents. While recent work has produced agents proficient in abstract games, these environments are far removed from the real world and thus these agents can provide little insight into the advantages of embodied play. Hiding games, such as hide-and-seek, played universally, provide a rich ground for studying the impact of embodied gameplay on representation learning in the context of perspective taking, secret keeping, and false belief understanding. Here we are the first to show that embodied adversarial reinforcement learning agents playing Cache, a variant of hide-and-seek, in a high fidelity, interactive, environment, learn generalizable representations of their observations encoding information such as object permanence, free space, and containment. Moving closer to biologically motivated learning strategies, our agents' representations, enhanced by intentionality and memory, are developed through interaction and play. These results serve as a model for studying how facets of vision develop through interaction, provide an experimental framework for assessing what is learned by artificial agents, and demonstrates the value of moving from large, static, datasets towards experiential, interactive, representation learning.
grounded in the real world. This requires a fundamental shift away from existing popular environments and a rethinking of how the capabilities of artificial agents are evaluated. Our agents must first be embodied within an environment allowing for diverse interaction and providing rich visual output. For this we leverage AI2-THOR [26] , a near photo-realistic interactive simulated 3D environment of indoor living spaces, see Fig. 1a . After our agents are trained to play cache, we then probe how they have learned to represent their environment. Our first set of experiments show that our agents develop sophisticated low-level visual understanding of individual images measured by their capacity to perform a collection of standard tasks from the computer vision literature, such as depth [43] and surface normal [15] prediction, from a single image. Our second collection of experiments, created in analogy to experiments performed on infants and young children, then demonstrate our agents' ability to integrate observations through time and understand spatial relationships between objects [8] , occlusion [19] , object permanence [35] , seriation [35] of free space, and perspective taking [31] .
We partition the game of cache into five conceptually-distinct stages: exploration and mapping (E&M), perspective simulation (PS), object hiding (OH), object manipulation (OM), and seeking (S); see Figures 1b to 1f. Narratively, cache begins with the hiding agent exploring its environment and building an internal map corresponding to the locations it has visited (E&M). The agent then chooses globally, among the many locations it has visited, a location where it believes it can hide the object so that the seeker will not be able to find it (PS). After moving to this location the agent makes a local decision about where the object should be placed, e.g. behind a TV or inside a drawer (OH). The agent then performs the low-level manipulation task of moving the object in its hand to the desired location (OM), if this manipulation fails then the agent tries another local hiding location (OH). Finally the seeking agent searches for the hidden object (S). Pragmatically, these stages are distinguished by the actions available to the agent (see Table 1 ) and their reward structures (see Methods). These agents are parameterized using deep convolutional and recurrent neural networks (see Fig. 5 ), abbreviated as CNNs and RNNs respectively, and trained adversarially using the paradigms of reinforcement [30] and self-supervised learning. While methodology for reinforcement learning is not the focus of this work, designing a system allowing for the joint training of these complex and interdependent stages is non-trivial and benefits from several novel solutions to technical challenges, see Methods. We distinguish two means by which our agents represent their environment. The first, static image representations (SIRs), correspond to the output of a CNN applied to the agent's egocentric visual input. The second, dynamic image representations (DIRs), correspond to the output of the agents' RNN. While SIRs are timeless, operating only on single images, DIRs have the capacity to incorporate the agent's previous actions and observations.
The quality of SIRs is often measured by their capacity to predict a suite of standard physical and semantic features of a static environment [51] . We found that producing high quality SIRs using existing reinforcement learning techniques, such as A3C [30] , to be infeasible as the gradients produced by A3C suffer from high variance and so signal struggles to propagate through the many convolutional layers. To correct this deficiency we introduce a technique we call a visual dynamics replay (VDR) inspired by experience replay [29] , hippocampal replay, and research in intrinsic motivation for artificial agents [33] . In visual dynamics replay, agent state transitions produced during training are saved to a separate thread where SIRs are trained to predict a number Notice that in c the agent must choose where to hide the object at a high level, using its map of the environment to make this choice, while in d the agent must choose where to hide the object from a first person viewpoint. In e the object being manipulated is a tomato.
of targets including forward dynamics, predicting an image from the antecedent image and action, and inverse dynamics, predicting which action produced the transition from one image to another. Static tasks. To evaluate the quality of our SIRs we train a decoder model to predict, from the SIRs, geometry and appearance-based canonical computer vision targets including pixel accurate depth, surface normals, and object class. Additionally, we consider several affordance-based targets, namely traversable surfaces, object openability, and two tasks requiring inference about geometry behind an object (see Figures 2b to 2d for visual examples). On the test set, our SIR consistently outperforms an autoencoder baseline (p < 0.001 in all settings except for object classification where p = 0.88, see Fig. 2a ) and compares favorably to representations produced by a model trained on the 1.28 million hand-labelled images from ImageNet [12] , the gold-standard for fully-supervised representation learning with CNNs. Note that our SIR is trained without any explicitly human labeled examples.
While modern CNN models can perform surprisingly sophisticated reasoning on individual images, the representations learned by these models are fundamentally timeless and thus cannot Figure 2 : Evaluating the static image representations learned by our model. a Comparing SIRs learned by playing cache against two baselines, one trained to auto-encode images from AI2-THOR (unsupervised) and the other trained using ImageNet (fully supervised). We display test-set losses (averaged first within each scene) of the cache and the auto-encoder SIRs across multiple tasks and relative to the ImageNet SIR losses. Values less than one indicate performance better than that of the ImageNet SIR and a solid, as opposed to crosshatched, fill indicates that this difference is significant at a <0.01 level using a generalized linear mixed-model (GLMM), see Methods. b-d Qualitative examples of geometric and affordance-based image predictions using the cache SIR. Input images are from the held-out test set. hope to model human-level visual understanding which is enhanced by memory [49] and intentionality [11] . Through experiments inspired by those from developmental psychology we now show that such, memory enhanced, representations emerge within our agents' dynamic image representations (DIRs) when playing cache. When applicable our experiments are also run using DIRs from an agent trained to perform visual navigation, a task with an extensive history in the study of embodied visual agents [6] . Dynamic object-centric tasks. Developmental psychology has an extensive history of studying childrens' capacity to distinguish between categories of spatial relationships [36] . Recently, infants as young as six months have been shown to have the capacity to differentiate between an object being contained in, or behind, another object [8] . Following this line of work, we design an experiment to test our agents' ability to perform an analogous discriminative task. In this experiment the hiding agent is made to move an object through space until the object is placed within, or behind, a receptacle object (see Fig. 3a ). From the hiding agent's DIR of the object trajectory we then predict the relationship between the object and the receptacle using a linear classifier. So that we are not simply measuring our agent's capacity for memorizing visual features, our testing environment involves objects, receptacles, and scenes that were not available while training the linear classifier. We find that we can discriminate between contained/behind object relationships (accuracy of 68.44%, 95% confidence interval (CI) ±1.56%) and can do so significantly more accurately than when using SIRs alone (mean difference in percentage accuracy 13.16%, 95% CI ±0.091%); see Fig. 3a . We next consider another object-centric milestone in cognitive development, the understanding of object permanence.
Recognizing object permanence, that objects continue to exist even when not visible, is a first and necessary step as infants move from pure egocentrism towards a well-developed theory of mind [35] . Indeed, infants begin to appreciate object permanence at as early as two and a half months [2] highlighting the concept's importance as a building block of higher level cognition. We design two experiments to test our agents' understanding of object permanence. First, see Fig. 3b , we find that we are able to infer the location of an object on a continuous path after it becomes fully occluded with significantly greater accuracy when using the DIR of the hiding agent instead of a corresponding SIR (mean difference in accuracy 9.9%, 95% CI ±1.75%). Note that, despite the apparent lack of visual signal, it is important to compare against an SIR as a baseline as there are visual biases as to where the object could, in principle, be occluded. Second, see Fig. 3c , we test our agents' ability to remember and act on previously seen objects. We find that our seeking agents' DIR can be used to predict whether the agent has previously seen a hidden object even after the agent has been rotated by 180 • so the object is entirely outside its visual range (accuracy of 89.43%, 95% CI ±2.35%). In infants, there is substantial evidence that while very young infants are surprised by impossible occlusion events, showing an appreciation for object permanence, they struggle to act on this information until around nine months of age [19] . Surprisingly we see that our seeking agent is significantly more likely to rotate back in the direction of a previously seen hidden object (mean difference in log-odds of rotating back towards the object versus rotating in the other direction between treatment and control agents 0.14, 95% CI ±0.034) demonstrating the ability for the agent to act on its understanding of object permanence. Moving from object-centric visual understanding, we now examine our agents' understanding of space. Dynamic space-centric tasks. Piaget argues that the capacity for seriation, ordering objects based on a common property, develops after age seven during the concrete operational stage of cognitive development [35] . While we cannot hope that our agents have learned the refined understanding of seriation present in seven year old children we hypothesize that rudimentary seriation should be possible among properties central to cache. One such property is free space, the amount of area in front of the agent that could be occupied by the agent without collision, which is critical for successful navigation and exploration. To test the capacity of our agent to seriate free space, we place our agent randomly within the environment, rotate it in 90 • increments (counter-) clockwise, and save the corresponding SI and DI-representations after each rotation (see Fig. 3d ). We then ask the agent to perform a two-image seriation, namely to predict, from the representation saved at time t, whether the viewpoint at the previous timestep t − 1 contained more free space. Our agent's DIR representation is able to perform this seriation task with accuracy significantly above chance (mean accuracy of 83.99%, 95% CI ±2.54%) and does substantially better than the corresponding SIR (mean difference in accuracy 8.6%, 95% CI ±3.33%). Our agent's DIR encodes an understanding of relative free space through time and stores this information in a state that is readily accessible. We now evaluate the policy our agents have learned to play cache and, thereby, evaluate our agents perspective taking ability. Playing cache and perspective taking. We have shown that the DIRs learned by modern neural network models through playing cache encode several fundamental building blocks of higher-order Figure 3 : Evaluating the dynamic image representations learned by our model. Schematic illustrations of the experimental designs of our psychologically motivated tasks paired with examples of these tasks in AI2-THOR and numerical results. For the numerical results, we report test-set performance of SIRs and DIRs with 95% confidence intervals. See Methods for definitions of plot labels. Note that the x-coordinates in our line charts are offset slightly from one another so as to improve readability. As SIRs are obtained from individual images we specify how these individual images are chosen, when applicable, below. In each of these tasks a linear classifier is used to predict, from the SIR or DIR, the target of interest. Here we show the performance of the representations as the amount of data used in training the linear classifier increases, see Methods for details on this training procedure. In the main text, we report only the values corresponding to the largest training set sizes considered. a The agent observes an object being placed either inside, or behind, a receptacle object and must distinguish between these two cases. Here SIRs are formed using the final image from the object's trajectory. b The agent observes an object moving behind an occluder and must, at several time points, predict the object's location. SIRs are obtained from images where the object is fully obscured. c The seeking agent rotates away from a seen object in two 90 • increments and must distinguish this case from a paired example where the object was invisible. The solid and dashed lines in the line chart correspond to the agent's DIR after one and two 90 • rotations, respectively. d The agent stands in a room and rotates by 90 • increments. After each rotation the agent must predict if its current viewpoint has more free space than its prior viewpoint. SIRs are obtained from the agent's current viewpoint.
reasoning and theory of mind. Evidence suggests that in humans, perspective taking, the ability to take on the viewpoint of another, possibly hypothetical individual, develops well after infancy with a rudimentary competence beginning around age four [50] . While standard recurrent neural networks can perform surprisingly sophisticated tasks, there is little evidence to suggest they can perform the multi-step if-then reasoning necessary in perspective taking. Taking inspiration from the intuition guided Monte-Carlo tree search used within AlphaGo [47] and from imaginationaugmented RL agents [39] we require, in the perspective simulation stage, that our hiding agent evaluate prospective hiding places by explicitly taking on the seeker's perspective using internal mental rollouts (see Fig. 4c and Methods). Rather than evaluating this explicit perspective taking module alone, we follow work with children [31, 50] , where performance in hiding games is used as a holistic measure of perspective taking ability. That is, we use our agents' overall performance in cache as a proxy for perspective taking ability. Figures 4a to 4f give a qualitative overview of the policy our agents have learned for playing cache. As these figures show, our agents have learned to explore, navigate, provide intuitive evaluations of hiding spaces, and manipulate objects. Figures 4g to 4h, show quantitatively that hiding agents from later in training consistently chose hiding places that our seeking agents are less likely to find. Moreover, we see that for hiding spots generated by human experts and by an automated brute force approach, seeking agents from later in training more frequently find the hidden objects. Interestingly, those hiding spots chosen by humans appear to be as difficult to find as those generated by a brute force approach with the best seeking agent only finding these objects in 37.3% of cases (on the test set, in comparison to 68.15% for those hiding places generated by our best hiding agent). Surprisingly, as shown in Table 4i , human seekers find objects hidden by humans and by our hiding agent at similarly high rates (≈80% of the time). Taking a nuanced view, this table provides some evidence that human seekers take fewer steps to find objects hidden by our artificial agent than those hidden by other humans, as measured by median number of steps taken to find the object, but these differences are not statistically significant. Taken together, this suggests that while there is substantial room for improvement before our artificial agents' perspective taking ability rivals that of humans, adult humans' high competency in object seeking makes these differences difficult to detect with purely human evaluation. c, a hiding location for the object must be chosen in pixel space. Here the first person view of the agent is paired with two sets of heatmaps. The top row shows the probability, from an SIR, that the object can be placed in a location. The bottom row shows the probability, averaged over the episode, of our agent trying to hide the object in a location. e The agent attempts to move the object to the location requested in d. The object's trajectory through space is shown as the agent successfully moves it to lie between two trash cans. f A top down view of the trajectory of the seeking agent. Initially it explores in the incorrect direction but eventually finds the object. g,h Frequency that seeking agents from three points during training (after approximately 0, 2•10 5 , and 2•10 6 episodes of training) find objects hidden by various hiders. Hiding agents include those from the same three points during training, those generated by an exhaustive approach (E=easy, M =medium, H=hard), and hiding spots chosen by humans. Error bars denote 95% CIs. i Results of our best hider (h 2e6 ) and seeker (s 2e6 ) competing with humans. Here the mean frequency of finding the hidden object is reported (with 95% CIs) along with the median number of steps the seeker took to find the hidden object (with 95% bootstrap CIs).
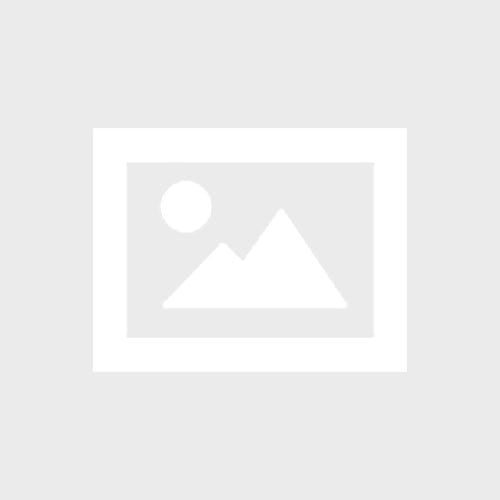
Modern paradigms for visual representation learning, generally requiring large human-labelled datasets, have led to startling performance on a large suite of tasks such as object recognition and detection. While these methods have revolutionized computer vision, it is difficult to imagine that they alone will be sufficient to learn visual representations mimicking humans' capacity for visual understanding which is augmented by intent, memory, and an intuitive understanding of objects and their physical properties. Without such visual representations it seems doubtful that we will ever deliver one of the central promises of artificial intelligence, an embodied artificial agent who achieves high competency in an array of diverse, demanding, tasks. We believe that it is time for a paradigm shift. Visual representation learning should move away from large, static, datasets and towards experiential, interactive, learning. Our experiments, in showing that rich interactive gameplay can produce representations which encode an understanding of containment, object permanence, seriation, and perspective taking, suggest that this direction is promising. Gameplay inspired by children and animals is a means by which we may build artificial models more closely mimicking biological agents in their ability to reason about their environment through time. Moreover, we have shown that experiments designed in the developmental psychology literature can be fruitfully translated to understand the growing competencies of artificial agents which, as these agents become increasingly complex, quickly outpace our ability for direct inspection.
s,o , S med. pos. s,o , and S hard pos. s,o. To define these collections
. Opening objects before placing things into them: we designed a loss that encouraged the agent, during the OM-episode, to try opening an object whenever the goal location for the object was in a CONTAINEDIN modality.3. Predicting if a goal object location was attainable: for better sample efficiency and for use during other stages, we encourage the agent to predict whether or not it will be able to manipulate the current object in such a way that it will reach the given goal object location. In particular, assume that the agent was directed to place the object in location (m, i, j). The hiding agent then
return r
Methods
Virtual environment and preprocessing While AI2-THOR can output images of varying dimensions and quality we find that simulating images of size 224 × 224 with the "Very Low" quality setting provides a balance of image fidelity and computational efficiency. AI2-THOR provides RGB images with the intensity of each channel encoded by an integer taking values between 0 and 255. Following standards used for natural images, we preprocess these images by first scaling the channels so that they are floating point numbers between 0 and 1 after which we normalize the R, G, and B channels with means 0.485, 0.456, 0.406, and standard deviations 0.229, 0.224, 0.225. Agents are constrained to lie on a grid with 0.25 meter increments and may face in one of four cardinal directions with 0 • being north and 90 • being east. The agent's camera is set to have a field of view of 90 • , is fixed at an angle 30 • below the horizontal, and lies at a height of 1.5765 meters when standing and 0.9015 meters when crouching. Following conventions in computer graphics, the agent's position is parameterized a single (x, y, z) coordinate triple with x and z corresponding to movement in the horizontal plane and with y reserved for vertical movements. Other than when standing and crouching, our agent's vertical position is fixed. Letting Z denote the integers, an agent's position is uniquely identified by a location tuple
(x, z, r, s) ∈ (0.25 • Z) × (0.25 • Z) × {0, 90, 180, 270} × {0, 1}
where x, z are as above, r denotes the agent's rotation in degrees, and s equals 1 if and only if the agent is standing. It will be useful in the following to be able to easily describe subcomponents of a location tuple, to this end we let proj −x : R 4 → R 3 be the projection removing the first coordinate of a location tuple so that proj −x (x, z, r, s) = (z, r, s), and similarly for proj −z , proj −r , proj −s . AI2-THOR contains a total of 150 scenes evenly grouped into five scene types, kitchens (1-30), living rooms (201-230), bedrooms (301-330), bathrooms (401-430), and foyers (501-530). Excluding foyers, which are reserved for our dynamic image representation experiments and used nowhere else, we consider the first 20 scenes of each scene type to be train scenes, the next five of each type to be validation scenes, and the last five of each type to be test scenes. When training our cache agents we only use kitchen and living room scenes as these scenes are relatively large and often include many opportunities for interaction. Code and data availability On publication all code and datasets analyzed and generated during the present study will be made open source and freely available online. As our code will be made available, we will, in the below, omit the many minor implementation details that have gone into our experiments.
Cache Stages
In cache the hiding agent, or hider, attempts to place a goal object in a given AI2-THOR scene such that the seeking agent, or seeker, cannot find it. This goal object is one of five standard household items, see Fig. 6 , being either a loaf of bread, cup, knife, plunger, or tomato. Recall that we divide the game of cache into the five stages S stages = {E&M, PS, OH, OM, S}. The hider participates in the first four of these stages and the seeker in the last stage. Each of these stages is associated with a distinct discrete collection of possible actions, see Table 1 . When a stage s ∈ S stages is instantiated, so that the scene has been chosen and all goals specified, we call this instantiation an s-episode. During an s-episode, an agent takes actions, each taking a single unit of time called a step, until the goal state has been reached or a maximum number of steps, N max steps s , has been taken. Episodes begin with time t = 0 and t advances by 1 after every action regardless of whether or not that action is successful. Note that these episodes must be sequentially instantiated and we call a collection of sequentially instantiated episodes ending with an S-episode a game of cache. In the following we describe these stages in detail along with the metrics we use to track the quality of the learned policies and assign rewards. In order to describe these metrics we require the following sets. First let S visited
H,t (S visited S,t
) be the set of all location tuples visited by the hider (seeker) by time step t of its episode. Similarly we let S opened
H,t (S opened S,t
) be the set of all objects the hider (seeker) has opened by time step t. Finally we let S extrap.
H,T
be the set of all location tuples that can, assuming the agent can move through objects, be reached by starting at some location in S visited H,t and moving the agent ahead by 0.25m and then either staying put, moving left by 0.25m, or moving right 0.25m. Exploration and mapping (E&M) A game of cache begins with an E&M episode. During this episode, the agent may traverse the scene and interact with objects while building a representation of its environment for up to N max steps E&M = 200 steps. In our implementation, this representation is a metric map, see the model architectures section below, which is then available in the subsequent PS-and OH-episodes. Let S reachable be the set of all feasible location tuples in a given episode and, similarly, let N openable be total number of objects that can be opened. We evaluate the quality of exploration with three metrics:
Q coverage = |proj −r (S visited H,200 )|/|proj −r (S reachable )|, Q coverage+ = |proj −r (S extrap. H,200 ) ∩ proj −r (S reachable )|/|proj −r (S reachable )|, and Q open% = |S opened H,200 |/N openable .
Q coverage and Q coverage+ evaluate the agent's ability to traverse the entire scene while Q open% evaluates how frequently the agent interacts with its environment. Perspective Simulation (PS) After exploration the hider is allowed to consult the representation it has developed of its environment and must, from that representation, pick a single location tuple to which the agent will then be moved. As we do not give the agent a global reference frame it must choose this location tuple by specifying a location relative its final position after the E&M episode.
As the hiding agent is not constrained to pick a valid location tuple, it may, for instance, choose a location that is outside of the scene or already occupied by an object, such a choice is considered a failed action. As the quality of a choice of location tuple in this episode is tied intimately with the outcome of the OH-episode we will measure the progress of training for both episodes using the same metrics described in the section below.
Object hiding (OH) In the OH-episode the agent has been moved to a location tuple determined in the PS-episode and is now holding the goal object. In this episode the agent is not allowed to perform any navigational actions beyond standing or crouching, but may interact with objects by opening or closing them. Most importantly, the agent must choose a location in its current view at which it should hide the item. To discretize the possible hiding locations recall that the images available to agents are of size 224 × 224. Viewing C = {1, ..., 224} 2 as a grid of pixel coordinates, let (C ij ) 1≤i,j≤7 be a partitioning of C into a 7 × 7 grid so that
C ij = {(32 • i + k, 32 • j + l) | 1 ≤ l, k ≤ 32} is of size 32 × 32.
When specifying a location in which to hide the object, the agent picks one of these grid locations along with one of the positional keywords in the set S modality = {ONTOP, CONTAINEDIN, BEHIND}. After choosing a location we begin an OM-episode, described below, during which the agent attempts to move the object through space and to the specified location. In order to reduce the complexity of specifying plausible object locations, potentially difficult for instance when specifying a final location behind another object, we consider an object to have been successfully placed at the grid location C ij , when using the CONTAINEDIN, BEHIND keywords, even in those cases that it moved to
C (i−1)j , C (i+1)j instead.
If OM-episode is successful given this relaxation, all future actions specifying object locations are considered failures. If the OM-episode is unsuccessful the hiding agent may continue to specify locations, causing the item to be picked up and a new OM-episode to begin, until manipulation is successful. If the hiding agent fails to successfully place the object within N max steps OH = 15 steps the object is returned to its default position in the agent's hand and then simply dropped. Otherwise, the agent may continue to take other actions, closing a cabinet into which it has placed an object for instance, or specify that it is done by performing the ReadyForSeeker action. Objectively quantifying a hiding location is difficult as the quality of a location is coupled with psychology and artifacts of perception. Indeed, the phenomenon of an object being lost "in plain sight" suggests that seemingly irrational hiding strategies may be highly successful. Nevertheless, we require some automated metric with which to measure the quality of a hidden object and, for this purpose, define the following three metrics. First we let N visible from be the number of feasible location tuples from which any portion of the goal object can be seen at any distance. To complement N visible from with a measure that takes the seeking agent's start location into account, we let N BFS steps be the number of steps it takes a breadth first search (BFS) agent, starting at the seeking agent's start location, to find the goal object. A single step for the BFS agent corresponds to teleporting to the location tuple closest to the seeker's start position that has not yet been visited by the BFS agent and checking if the goal object can be seen from any rotation, crouching or standing, from that location. The BFS agent is allowed to see through openable objects and the goal object is considered found if any portion of the object can be seen at any distance. If the BFS agent cannot find the object then N BFS steps equals the maximum number of steps, |proj −r (proj −s (S reachable ))|, in which case we let δ BFS found object equal 0, otherwise δ BFS found object equals 1. Finally we also define the proportional versions of two of the above metrics.
Q visible from% = N visible from /|S reachable |, and
Q BFS steps% = N BFS steps /|proj −r (proj −s (S reachable ))|.
Object manipulation (OM) In the object manipulation episode the agent is set at a fixed location tuple holding the goal object and is given a grid location 1 ≤ i, j ≤ 7 along with the positional keyword MODALITY ∈ S modality . During this episode the agent can interact with objects by opening them, move the object held in its hand in one of six axis-aligned directions, or drop the goal object. The object manipulation episode is considered successful if, after dropping the goal object, the goal object is in the correct grid position specified by i, j and respects the constraints imposed by MODALITY. In particular, after the manipulator has dropped the object, we obtain from AI2-THOR the sets S ONTOP pix. = {coordinates of pixels corresponding to the object that are visible from the agent's current viewpoint}, S BEHIND pix. = {pixel coordinates of the object that are visible only after making all other objects in the scene invisible}, and the value δ CONTAINEDIN ∈ {0, 1} which equals 1 if and only if the goal object is contained within another object. If MODALITY = ONTOP then the episode was successful if δ CONTAINEDIN = 0 and |C ij ∩ S ONTOP pix. | ≥ 10, i.e. if at least 10 of the object's pixels lie in the correct location and are visible. Similarly if MODALITY = BEHIND then the episode was successful if δ CONTAINEDIN = 0 and |C ij ∩ S BEHIND pix. | ≥ 10. Finally if MODALITY = CONTAINEDIN then the episode was successful if δ CONTAINEDIN = 1 and |C ij ∩ (S ONTOP pix. ∪ S BEHIND pix. )| ≥ 10. To track the progress of this episode we simply record successes, namely we let δ hit target ∈ {0, 1} equal 1 if and only if object manipulation was successful. Note that, in the OH-stage, a OM-episode is considered successful even if δ CONTAINEDIN = 0 and the modality is ONTOP or BEHIND. Seeking (S) In the seeking episode the seeking agent is tasked with finding the object hidden by the hiding agent. The seeking agent begins from the same location tuple as the hiding agent began in the E&M-episode and given a budget of N max steps S = 500 steps before the episode is considered a failure. During these 500 steps the seeking agent may perform any of the actions allowed in the exploration and mapping episode along with one additional action, ClaimVisible. If the seeking agent performs the ClaimVisible action successfully the seeking agent has successfully found the goal object and the episode ends. Just as when attempting to automatically measure the quality of a hiding location, developing objective measures of the quality of a seeking policy is challenging. As described previously, a seeking strategy that is highly successful against one hiding strategy may be fatally flawed when faced with another. To remove any dependence on a hiding agent obtained through reinforcement learning we generate, through an exhaustive, brute force approach, three collections of hiding locations for each scene s and object o, S easy pos.
we use AI2-THOR to, for each goal object o and scene s, generate a collection
L o,s = { o,s,i | 1 ≤ i ≤ N o,s } of N o,s
0 possible hiding locations. For each o,s,i we compute v o,s,i which equals the Q visible from% metric defined above for the given hiding location. , is then a randomly sampled collection of, approximately, 20 easy locations from L o,s . We then quantify the quality of a seeking policy by computing the success rate of the seeker when the hiding locations are restricted to those from each of the above three sets.
Letting V o,s = {v o,s,i | 1 ≤ i ≤ N o,s },
Training And Losses
We train using eight GPUs with one GPU reserved for running AI2-THOR processes, one reserved for VDR, and the other six dedicated to training with reinforcement and self-supervised learning. Each of the cache stages generates its own losses, detailed below, which are minimized by HOG-WILD! [40] style unsynchronized gradient descent to train model parameters. Reinforcement learning in the E&M, OH, OM, and S stages is accomplished using the asynchronous advantage actor-critic (A3C) algorithm [30] with generalized advantage estimation (GAE) [44] . For the A3C loss we let the discounting parameter γ = 0.99 (except for in the OH-stage where γ = 0.8), the entropy weight β = 0.01, and GAE parameter τ = 1. We use the ADAM optimizer [24] with AMSGrad [41] , moving average parameters β 1 = 0.99, β 2 = 0.999, a learning rate of 10 −3 for VDR, and varying learning rates for the different cache stages (10 −4 for the E&M, OH, OM, and S stages, 5 • 10 −4 for the PS-stage). In the below we give an overview of the self-supervised losses used during training as well as the reward structures necessary in the E&M, OH, OM, and S stages for computing the A3C loss. Visual dynamics replay Here we give an overview of our VDR training procedure. We direct anyone interested in exact reproduction of our VDR procedure to our code base. During training our agents save triplets (i 0 , a, i 1 ), where i 0 is an antecedent image, a is an action taken (with associated success or failure signal), and i 1 is the subsequent image resulting from taking action a when the agent's view was i 0 , to a queue that is then periodically emptied and processed by the VDR thread. The VDR thread processes the triplets by saving them into a buffer, similar to an experience replay buffer [29] . To ensure that certain actions do not dominate the buffer we group and selectively subsample the triplets added to the buffer. Moreover, the buffer automatically removes triplets to ensure that triplets do not exceed some fixed age and that the buffer does not exceed its max size of approximately 20,000 triplets. After processing incoming data and storing triplets in the dynamics buffer, the VDR thread then randomly partitions the buffer into batches of size 64 and, for each batch, computes the selfsupervised losses detailed below and performs a single gradient step to minimize the sum of these losses. We call a single pass through all batches an epoch. VDR then alternates between processing incoming triplets, forming batches, and training for a single epoch. The losses minimized in VDR include the following.
• Inverse dynamics loss: predicting a from i 0 and i 1 . From the VDR model we obtain, from i 0 and i 1 , a vector v inv. dyn. of length N actions = the total number of unique actions across all agents. By assigning each action a a unique index, index(a) ∈ {1, ..., N actions }, we then compute the inverse dynamics loss as the negative cross entropy between the point mass distribution δ index(a) and softmax(v inv. dyn. ).
• Forward dynamics loss: predicting i 1 from a and i 0 . From the VDR model we obtain, from i 0 and a, a tensor i n×n 1 ∈ R 3×n×n for n ∈ S = {14, 28, 56, 112, 224} where i n×n 1 represents the prediction of i n×n 1 which equals i 1 downsampled to have spatial resolution n × n. Lett ∆ n be the, per pixel, 1 error between i n×n 1 and i n×n
1
, and let ∆ n be its mean. We let the forward dynamics loss equal n∈S ∆ n . In practice we found it helpful to compute ∆ n as a weighted average with larger weight given to areas of the image that have changed.
• Pixel difference loss: predicting which pixels in i 1 are different from those in i 0 from i 0 and a. Computed similarly to the forward dynamics loss using a tensor T pix. diff. returned from the VDR model except using a binary cross entropy loss to predict which pixels are different between i 0 and i 1 .
• Pixel quality loss: predicting confidence of predictions of forward dynamics. From the VDR model we obtain a 1 × 224 × 224 tensor T confidence . We compute the pixel equality loss as the mean squared error between T confidence and ∆ 224 .
• Action success loss: from a and i 0 predict if the action would be successful. From the VDR model we obtain, from a and i 0 , T would succeed . The action success loss is then computed as the binary cross entropy loss between T would succeed and the indicator δ action did succeed .
Note that we only compute the forward dynamics loss and pixel quality loss on those triplets whose action a is a movement action, that is an action from Table 1 whose name includes the word Move or Rotate.
Training perspective simulation In this section, when we refer to a location tuple, we mean a location tuple without the standing component. Recall that in the PS-stage the agent must consult its map of the environment and, from this map, choose a location tuple where from which it will begin the OH-stage. In the following we describe our implementation of the PS-stage. We begin by describing the two-phase process by which a location tuple is chosen and then describe the losses we use to train this process. In the below description it will be helpful to define the set
S OH outcomes = {fail} ∪ ({0, 1} × {0, 1, 2} × {1, ..., 7} 2 )
where fail ∈ S OH outcomes denotes the event in which the OH-episode did not successfully choose a hiding location (so the object was simply dropped) and (s, m, i, j) ∈ S OH outcomes denotes the event in which, during the OH-stage, the agent successfully hid the object and
• the hiding agent was standing when it hid the object if s = 1 and was otherwise crouching,
• the hiding agent hid the object with a PlaceAt|m,i,j action.
For simplicity, we will say an object was hidden at ( , e) to mean that, during the OH-stage, the agent was located at and e ∈ S OH outcomes occurred. We now describe the two phase process for choosing a location tuple. Fig, 4b ). The agent assigns a score to all location tuples with the goal of highlighting locations that deserve further evaluation. This score, for a particular location tuple , is computed as the weighted average
1. Initial Evaluation (Recall
e∈S OH outcomes softmax(P ) e • V e
where P and V are generated by the agent and are described below.
2. Mental rollout (recall Fig. 4c ). The agent begins by picking the five highest scoring location tuples { 1 , ..., 5 } from the previous phase along with five randomly selected location tuples { 6 , ..., 10 }. For each 1 ≤ i ≤ 10, the seeking agent simulates the outcome of running the OHstage with the agent at at location i three times. Namely the agent iteratively samples three distinct elements e i1 , e i2 , e i3 ∈ S OH outcomes with the probability of sampling e ij ∈ S OH outcomes at every iteration being proportional to softmax(P i ) e i j .
Then, for each 1 ≤ i ≤ 10 and 1 ≤ j ≤ 3, the agent simulates 50 rollout trajectories (t ijk ) 50
K=1
of the seeking agent in the hypothetical setting in which the object was hidden at ( i , e ij ).
Let
µ ij = 1 50 50
k=1 #steps(t ijk ) be the average number of steps taken in the simulated seeker trajectories (t ijk ) 50
k=1 . Straightforward analysis, using the probabilities defined in softmax(P i ), shows that there is closed-form solution for weights w ij so that µ i = 3 j=1 w ij µ ij is an unbiased estimator of the expected mental rollout path length when hiding at ( i , e) and e is selected from S OH outcomes with probability softmax(P i ) e .
After computing µ = (µ 1 , ..., µ 10 ), the location tuple i where the actual OH-episode will begin is chosen by sampling i from a Multinomial(softmax(0.04 • (µ 1 , ..., µ n ))) distribution.
We now describe the losses minimized so that we obtain high quality initial evaluations of location tuples and mental rollouts of seeker trajectories. Given a tensor M encoding the agent's map of the environment (see the Metric mapping section below), the perspective simulation model produces an intermediate representation of this map M 1 . From M 1 it generates two tensors P and V of size 2×3×7×7 as well as two values p none , v none ∈ R. For simplicity we will abuse notation and let P none = p none and V none = v none . Now softmax(P ) e is a prediction of the probability that, were the agent to choose to hide the object at location , the event e ∈ S OH outcomes would occur. This tensor softmax(P ) is supervised using a crossentropy loss with the hiding location chosen during the OH-stage. The entries of the tensor V then represent an estimate of the quality of a hiding location. The collection of tensors V is supervised as follows. Let 1 ≤ i = i ≤ 10 and 1 ≤ j = j ≤ 3. Now let L V ij,i j be the binary cross entropy loss between V i e ij − V i e i j and sign(µ ij − µ i j ). Note that minimizing L V ij,i j encourages V i e ij to be larger than V i e i j if and only if µ ij > µ i j , namely in those settings where mental rollouts suggest that ( i , e ij ) is a better hiding location than ( i , e i j ). We thus let our loss supervising the tensors V equal the average L
V = 30 2 −1 sum({L V ij,i j | 1 ≤ i ≤ i ≤ 10, 1 ≤ j ≤ j ≤ 3, (i, j) = (i , j )}).
Now we discuss losses used to guide learning to produce mental rollouts of seeker trajectories. Suppose that a full game of cache has taken place. We begin by collecting several pieces of data from the game.
1. The location tuple during the PS-episode and the event e ∈ S OH outcomes that occurred during the OH-stage.
2. The trajectory (t i ) n i=1 (ordered set of location tuples visited) by the seeker.
3. A {0, 1}-valued vector v would succeed with ith entry equalling 1 if and only if the seeker would have been able to successfully claim the object was visible at the ith step of its trajectory.
4.
The actions a i taken by the seeking agent taken at step i along with their success signal s i .
5. The policy, probability distribution over actions, π i of the seeker at step i.
The hiding agent then forms, from the intermediary representation M 1 and pair ( , e), a hidingplace-conditional representation M ,e . Using this conditional representation as a "mental environment," the agent then simulates an internal seeker who followed the same trajectory (t i ) n i=1 and took the same actions (a i ) n i=1 as the true seeking agent. At every time step i the hiding agent generates a mental policyπ i for the simulated seeker along with a vector of probabilitiesŝ i whereŝ ij denotes the predicted probability that a seeking agent, at the location t i , taking action with index j would succeed in doing so. For every i, the mental policy π i is then trained, using a cross entropy loss, to mimic the true seeker policy π i . Similarly at every step, the predictionsŝ ij of whether actions would, or would no succeed, is supervised using a binary cross entropy loss with s i if j is the index of action a i . Furthermore eachŝ ij corresponding to the ClaimVisible action is supervised binary cross entropy with v would succeed . Additional losses To improve training sample efficiency we augment the usual A3C losses, described below, by also computing several other losses, these losses are averaged across timesteps within each stage and then summed together along with any other losses, e.g. the A3C loss, and gradients are computed for this total loss before being synchronized with the shared model. Let s ∈ S stages , t ≥ 0 be the current time step in s-episode, and i t be the image observed at that time step, if applicable. If s ∈ {E&M, OH, OM, S} and t > 1 then, as described in the neural models section below, at every time step the agent generates a 1 × 7 × 7 tensor W s,t where softmax(W s,t ) 1,i,j should is encouraged to be nonzero only if the pixels corresponding to C ij have changed from the previous to the current frame. To supervise W s,t we first generate the 1 × 7 × 7, {0, 1}-valued, tensor D where D 1,i,j equals one if and only if the pointwise difference between the encodings of i t and i t−1 generated by the first convolutional block of the PVCNN, has 10% of the spatial locations corresponding to the i, j grid location with 2 -norm greater than 10 −3 . We then form the loss
L = − log( 7 i=1 7 j=1 softmax(W s,t ) 1,i,j • D 1,i,j ).
Note that minimizing L encourages the sum of the entries in softmax(W s,t ) corresponding to entries in D equalling one to be large. We also include several stage-specific losses described below. As there are several stage specific losses we will not describe the full mathematical details of these losses and instead describe their intent. OH-specific losses.
1. Avoiding early PlaceAt modality collapse: early in training the hiding agent makes many failed PlaceAt actions, as a large number of these actions are impossible for a given viewpoint, incurring a substantial penalty for these repeated failures. Because of this, we found that, in this stage, the agent often would often quickly learn to only use the ONTOP modality of the PlaceAt as this is the modality is, generally, the easiest for the manipulator to successfully complete. To prevent this policy collapse, we introduce a loss that encourages the agent to maintain probability mass on PlaceAt actions with CONTAINEDIN and BEHIND modalities. This is similar to the standard loss in A3C encouraging higher entropy in an agent's policy. This loss is removed after 5,000 episodes of training.
2. Avoiding early PlaceAt spatial collapse: similarly as above, we sometimes found that the agent's policy would concentrate on PlaceAt actions at a single grid position i, j early in training. To prevent this, we create a loss encouraging the probability mass over PlaceAt to be similar to a prediction of which PlaceAt actions would be successful coming from the manipulator model (described below). As above, this loss is removed after 5,000 episodes of training.
3. Recalling PlaceAt actions: to encourage the agent to remember where it attempted to place the object and where, on those attempts, the object ended we designed two losses penalizing the agent for failing to accurate make such predictions correctly.
4. Closing objects: after placing an object so that is in the CONTAINEDIN modality we design a loss encouraging the agent to attempt closing objects.
OM-specific losses.
1. Better sample efficiency: inspired by hindsight experience replay [3] , we design a loss that rephrases failed trajectories made during an OH-episode, i.e. a trajectory that placed the object in a location that was not specified as the goal location, into a successful trajectory where the goal was whichever location the object actually was placed in.
produces, at every step, a 3 × 7 × 7 tensor T hittable, t and, at the end of the episode, computes an average binary cross entropy loss between the entries T hittable, t m,i,j and the indicator δ OM-episode success .
PS-specific losses. See the section on training perspective simulation above. S-specific losses.
1. Better sample efficiency through self-supervised imitation learning: using the mental representation of the environment created by hiding agent in the PS-stage we compute a shortest path from the seeking agent's current location to a location from which, in this mental representation, the object is visible and then encourage the seeking agent to follow this path.
2. Reducing false claim visible actions: at every step made by the seeker we encourage (or discourage) the agent from taking the ClaimVisible action based on whether or not the goal object is currently visible to the agent.
Reward structure for reinforcement learning In order to compute the A3C loss we must define, for each stage s ∈ S stages , a reward structure, that is, a function which assigns a reward r ∈ R to every state-action-state triplet (s, a, s ), where s denotes a possible agent state and a an action which, when taken from state s, resulted in the agent moving into state s . When applicable and unless otherwise stated, agents obtain a penalty of r step = −0.01 on every step to discourage long trajectories and an additional penalty of r fail = −0.02 whenever an action fails (for instance, when running into a wall). For clarity of exposition we will define the reward structure by defining r in the case that agent starts at a generic state s at time t ≥ 0 and performs action a resulting in a transition to state s . E&M reward structure. During exploration and mapping, we would like to encourage the agent to comprehensively explore the environment, including interacting with and opening objects if possible, with particular attention being paid to areas that would make good hiding locations. To this end, but for r E&M, hide,t described below, we define the E&M reward structure in Algorithm 1. Now for 0 ≤ s ≤ t + 1, at time s let m s be the metric map generated by the agent, s be the location tuple of the agent, and let v s be the value of the position s when evaluating m s using the perspective simulation module. Now letting unseen s = δ{with action a s the agent opened an object, or entered a location, for the first time}, we define
v t = ( t s=0 v s • unseen s )/( t s=0 unseen s )
so that v t captures an average quality of unique states the agent has visited up to and including time t. We then define the reward
r E&M, hide,t = 0.2 • min(1, max(0, v t+1 − v t )) • unseen t+1
Note that r E&M, hide,t will be 0 if the agent has not opened a new object or entered a previously unvisited location tuple and, otherwise, will only be large if the perspective simulation module considers the current state to be substantially more valuable than previously visited states. Thus r E&M, hide,t provides a signal through which the exploration agent may learn to visit states that the perspective simulation module considers good hiding locations. OH reward structure. Except for the definition of r OH, percentile , the reward structure for the OH episode is defined in Algorithm 2. In the below we define and motivate r OH, percentile . Suppose that the during the OH episode the hiding agent has successfully completed a PlaceAt action, we would like the reward given to the agent after its final action to reflect the quality of the hiding location chosen and thus a natural reward for this final action is number of steps taken by the seeker to find the object N S steps normalized by the maximum number of steps allotted to the seeking agent (500), i.e. the reward r S steps = N S steps /500.
Unfortunately r S steps fails in two respects.
1. The reward r S steps suffers from very high variance. As an example, consider the case in which the object is hidden directly behind the seeker. If the seeker performs two RotateRight actions it will immediately find the object but, if it instead chooses to search the area ahead of itself then it may be many steps before it backtracks to its starting position and finds the object.
In such a case the reward r S steps may be very large or very small with high probability.
2. The number of steps required to find the object is not only determined by the quality of the hiding place chosen in the OH episode but also by the topology of the scene and the location tuple chosen in the perspective simulation episode. Namely, in a large, maze-like, room the OH episode may terminate with the object in the open from the hiding agent's perspective (a poor hiding place) but still require many steps from the seeker to find the object as the location tuple chosen in perspective simulation was far away from the seeker's starting position.
This high variance and confounding with the quality of perspective simulation results in r S steps providing a poor signal as to where an object should be hidden in the OH episode. While the high variance of the reward is intrinsic to the game of cache, especially so in the case of randomly initialized agents, we can partially address the second issue above by considering a transformation of r S steps defined as follows. First we generate 100 sample seeker trajectories (s i ) 100 i=0 when simulating hiding the object at the location chosen during the PS-stage; see the training perspective taking section above for details as to how these trajectories are generated. Letting i be the length of s i , i.e. the number of steps the seeker took to find the goal object in that mental rollout, we then define
r OH, percentile = −1 + 2 • 1 100 100 i=1 δ [ i ≤ N S steps ] .
Assuming these mental rollouts are of reasonable quality, r OH, percentile naturally calibrates the reward for the topology and size of the scene. This helps disentangle the final reward in the OH episode from contributions made purely during the PS episode. OM reward structure. The OM episode runs for a maximum of 50 steps and has the reward structure for this episode is outlined in Algorithm 3. Broadly speaking, we reward the agent for dropping the goal object near the target location with proximity measured in the image space and for placing the object in a location respecting the target modality among ONTOP, CONTAINEDIN, and BEHIND. We found it especially important to give the agent a large negative reward whenever it placed the object outside of the agent's viewport. S reward structure. The reward structure of the seeking episode is simple and encodes our desire for the agent to locate the object in as short as time as is possible without having a large false positive rate, that is without having many instances in which the agent claims to be able to see the object when the object is not actually visible. This reward structure is given in Algorithm 4.
Pretraining the E&M and OM stages As the ability to navigate about a room and perform object manipulation are basic competencies for the hiding agent in cache, we pretrain our hiding agent on the E&M and OM-stages until it attains reasonable proficiency. The reward structure when training the E&M-stage was essentially identical to that from Algorithm 1 but without the r E&M,n hide,t reward, this trained for approximately 85,000 episodes. To pretrain the OM-stages we generated, across AI2-THOR scenes, a collection of plausible locations the objects could be placed when at location tuples, and then trained the hiding agent to attempt placing objects in these locations using the reward structure in Algorithm 3; the manipulator agent was pretrained for approximately 2.5 million episodes. We ran this pretraining procedure for approximately three days.
Neural Models
Here we give an overview of the neural network architectures we use to parameterize our agents. As these architectures can be complex, we do not attempt to make this description sufficiently comprehensive to exactly reproduce our results; we instead direct any parties interested in extract reproduction to our open source code base. Our neural network architecture contains components that are shared among both the hider and seeker as well as components that are duplicated across multiple stages. Shared components include the CNN used to process input images, mapping components, and embeddings for actions, goal objects, and agent standing state. Information flow to policy and value Here we describe how, during the E&M-, OH, OM-, or S-episode, data flows through our agents' neural architecture to produce the agent's policy and value of the current state. Unless otherwise noted above or below, any component mentioned is unshared, that is, there is a unique copy of this component, with its own learnable parameters, for each of the four stages. Suppose the agent has received input corresponding to timestep t ≥ 0 in stage s ∈ S stages and must take an action. This input comprises the following.
• A 3 × 224 × 224 (channels × height × width) tensor corresponding to the image of the agents' current view. This tensor is processed by a 13-layer CNN, which we call the primary visual CNN (PVCNN), similar to the encoder of a U-Net [42] . This results in an output tensor of size 128 × 7 × 7.
• A non-negative integer specifying the agent's last action (including a 'none' action when t = 0) along with a 'success' or 'failure' signal indicating whether the agent's last action succeeded. This pair of inputs is used to index into an embedding matrix resulting in a 16-dimensional embedding vector.
• A non-negative integer specifying whether or not the agent is standing. This integer is also used to index into an embedding matrix resulting in a 4-dimensional embedding
• A non-negative integer specifying the goal object (i.e. object to hide or object to seek). This integer is also used to index into an embedding matrix resulting in a 16-dimensional embedding vector.
• The 257 × 7 × 7 dimensional hidden state h s,t−1 and cell state c s,t−1 corresponding to the output of the Grid Long Short-Term Memory Unit [23] (GLSTM), described below, from the previous time step. If t = 0 then h s,t−1 , c s,t−1 are tensors filled with zeros.
• (Excluding OM) The agents' current metric map of it's environment, this includes a 144dimensional vector for every unique location tuple the agent has visited.
• (OH only) If the last action taken in the OH-episode was a PlaceAt, an action embedding specifying the location location the OM-episode placed the object, e.g. if the OM-episode placed the object in the C ij grid location with modality BEHIND, then the agent obtains the embedding for a successful PlaceAt|2,i,j action. As an object can potentially land in many grid locations and modalities simultaneously, the chosen action corresponds to the location and modality containing the largest number of the object's visible pixels.
• (OM only) The modality m and grid location i, j where the object should be placed. This triple is used to slice into from a parameter tensor of size 3×5×13×13 retrieving a 5×7×7 tensor which is concatenated to the 128 × 7 × 7 tensor of visual features.
Except for in the OM-stage which does not include mapping, the above agents then read from their metric map using both an attention mechanism and a collection of convolutional layers resulting in an embedding of size 356. In the OH-stage the agent additionally applies a three layer CNN to the image tiled with the object embedding to produce a 3 × 7 × 7 tensor, recall T hittable, t , with (m, i, j) entry trained to predict whether or not the manipulator can successfully place the current object in the modality specified by m and the grid location specified by i, j. All of the above embeddings are then processed, if necessary, to lie on a 7 × 7 grid. For instance the 16-dimensional object embedding becomes a 16 × 7 × 7 tensor T where T :,i,j is a copy of the original embedding for each i, j. Once processed these tensors are concatenated together to form the tensor T rnn input s of size N rnn input channels
s,t × 7 × 7 for s ∈ S stages . The T rnn input s,t
tensor, along with tensors h s,t−1 and c s,t−1 defined momentarily, is then fed into a Grid Long Short-Term Memory Unit (GLSTM) [23] , a recurrent neural network, producing two tensors, a hidden state h s,t and a cell state c s,t , each of size 257 × 7 × 7. From the hidden state h s,t we then obtain the 256 × 7 × 7 tensorh s,t = h s,t 1:256,:,: and the 1 × 7 × 7 tensor W s,t = h s,t 257,:,: • τ s where τ s ∈ R is a learned temperature parameter. The matrix W s,t is trained, using self-supervision, to predict what portions of the input image have changed from the previously seen input image. Using learned linear layers applied toh s,t andh s,t W s,t along with sum and max-pooling, we then produce two sets of logits l marginal,s,t and l conditional,s,t . The values l marginal,s,t correspond to the probability of performing a particular action type, e.g. a navigational action or a PlaceAt|Behind action, while l conditional,s,t correspond to the probabilities of a particular action given the type. Factorizing the probabilities in this way allows the agent to, in principle, avoid some of the problems occurring when agents have large action spaces. The agent then computes the value of the current state by first sum-pooling h s,t W s,t along spatial dimensions to produce an vector of size 256 and then passing the result through a linear layer to produce the 1-dimensional output v s,t . Finally, in the E&M-and S-stages, the agents then write into their map, at a position defined by their current location tuple, a 128-dimensional vector extracted from one of the final layers of the primary visual CNN's processing of the input image along with the 16-dimensional object embedding and 16-dimensional action embedding. Metric mapping architecture Intuitively, our metric mapping architecture is designed so that an agent can record information regarding its current state for later retrieval. This map is called metric as data is written into the map so that it is spatially coherent, that is, where data is written in memory is uniquely defined by the agent's location tuple when it is written. Our metric map model implements is designed to allow for the following.
• Writing: given the current location tuple of an agent, passes a given value through a Gated Recurrent Unit (GRU) [10] whose hidden state is taken from the last value saved at that location (if any, otherwise a learned initial state is used) and this output of the GRU then overwrites the previously saved values.
• Reading at location: given a location tuple, returns the value saved at that location.
• Reading with attention: given an input key, uses a soft-attention mechanism to return a weighted sum of saved values. • Reading egocentric map: the same as reading as map but with spatial coordinates centered on the agent and appropriately transformed so that north is whichever direction the agent is currently facing.
Using the above, the agent can build a map while it explores its environment while querying that map locally and globally so as to make decisions.
Visual dynamics replay decoder architectures Recall from our training and losses section, that in VDR we are given a triplet (i 0 , a, i 1 ), where i 0 , i 1 are two images with i 1 occurring after an agent took action a, and must, accomplish a number of self-supervised tasks. To accomplish these tasks we require several neural network architectures building upon our primary visual CNN (PVCNN). These architectures are all convolutional neural networks with the largest of these networks is inspired by spatial transformer networks [22] , as well as U-Nets, and contains 13 convolutional layers, 10 of which are transposed convolutions which work to upsample the input tensor, from the PVCNN, to a final size of 16 × 224 × 224 after which 1 × 1 convolutions are used to producê
i 224×224 1
as well as T confidence and T pix. diff. . The tensor T would succeed is produced by a convolutional network acting on the PCVNN's embedding of i 0 and the embedding of a while the value v inv. dyn. is produced by a convolutional network applied to a concatenation of the PVCNN's embeddings of both i 0 and i 1 . Implementation details can be found in our code base. Perspective simulation architecture The perspective simulation architecture operates on the tensor representation of the map, see the metric mapping architecture above, which is reshaped to be of size 1152×h×w where h and w are the height and width of the map respectively. First this map is concatenated with a grid of distances from the agent start location after which it is processed by several 1 × 1 convolutional blocks to form the intermediary representation M 1 of the map. An additional 1 × 1 convolution produces, for each location tuple (merging those which only differ in that the agent is, or is not, standing) after and after reshaping, two tensors P and V of size 2 × 3 × 7 × 7 as well as two values p none , v none ∈ R. For simplicity we will abuse notation and let P none = p none and V none = v none . Next, given a hypothetical hiding outcome e ∈ S OH outcomes another convolutional neural network, acting on M 1 along with an embedding of e indicating where in M 1 the object is being hidden, produces the second intermediary representation of the map M 2 . A "mental" seeker agent is then defined in this architecture using an LSTM whose observations come from slices of M 2 . This mental seeker can move about the map defined by the representation in M 2 and, at each step i, produces the vectorsπ i andŝ i defined in the training in losses section by using two linear layers acting on the mental agent's hidden state.
Human And Self Evaluation For Cache Agents
In Figures 4g to 4i we report the results of evaluating our hiding and seeking agents from three points during their training as they compete against one another as well as against hiding places chosen by an exhaustive approach and hiding places chosen by humans. In the following we discuss how we collected hiding locations for the various agents and ran our seeking trials. Generating hiding locations Human agents. We recruited 16 human volunteers to hide objects within AI2-THOR. Participants were instructed that they would be randomly positioned in a simulated environment holding an object which they were then expected to hide so that a seeking agent, who started in the same position that they started in, would find the object difficult to find. Furthermore participants were instructed to be in the mindset that they might be playing against the same seeker many times and thus should choose diverse hiding locations. Before beginning, participants were allowed to familiarize themselves with the interface until they judged themselves sufficiently competent to begin the task. Unlike our artificial agents, humans subjects were not constrained in the number of actions they were allowed to take and, after hiding their object, were allowed to pick it up again and try other hiding locations if desired. We collected 600 human hiding locations evenly distributed among all object types and training, validation, and test scenes. To reduce the amount of data to be collected we used only ten (of 40) training scenes (AI2-THOR floor plans 7, 10, 13, 16, 18, 203, 209, 213, 215, and 218) . Artificial agents. We generate hiding locations from our artificial hiding agent using the agent after it had trained for 0, 210,000 and 2,355,000 episodes of cache. We call these agents h 0 , h 2e5 , and h 2e6 . For each of these agents we generate 4,000 total hiding locations, 2,000 each for the train and test scenes. The train (test) hiding locations are generated such that for each (scene, object type) pair we have an equal number of hiding locations. For fair comparison, we also generated a set of 1,545 additional hiding locations from h 2e6 using the same scenes, object types, and agent starting locations as those from the human trials (with multiple repeats per agent start location). Exhaustive approach. Recall the exhaustive automated approach for generating hiding places of easy, medium, and hard difficulties detailed in the cache stages section. Using this approach we generate, for each of these difficulties, 3,999 hiding locations in the train scenes and 998 in the test scenes. Because of our automated, metric-based, approach for generating these hiding spots there were three cases (one in the train scenes and two in the test scenes) where there were not be sufficiently many hiding locations 20 desired locations per object, scene, and difficulty. In such cases we reduced the number of locations saved for that object and scene pair for each difficulty level so that the total number of hiding places in each difficulty type remained equal. Running seeking trials In these trials a seeking agent is required to find an object hidden in a hiding location from one of the above collections. If, through automated checks, we find that a hiding place is such that the hidden object cannot be seen from any reachable position in the scene then this hiding location is never shown to any seeking agent and all seeking agents are considered to have failed to find the object for that trial. Artificial agents. We run seeking trials using the artificial seeking agents from the same three points in training and name these seeking agents analogously as s 0 , s 2e5 , and s 2e6 . Human agents. We again recruited 16 human volunteers to seek objects within AI2-THOR. Participants where instructed that they would be randomly positioned in a simulated environment and then need to find a hidden object. As in the hiding task participants were allowed to familiarize themselves with the interface until they judged themselves sufficiently competent to begin the task and were not constrained in the number actions they were allowed to take. On starting a trial, the participant was randomly assigned a hiding location generated either by h 2e6 or from the set of 600 human locations. To ensure fair comparison, we matched each of the 600 human locations to a unique hiding location generated by h 2e6 (from the additional set of 1,545) where the artificial hiding agent started the same position as the human hider. After 30 seconds participants were allowed to give up, moving them to the next seeking trial, if they felt the object could not be found. At the end of these trials we were left with complete seeking trials for 595 human-generated hiding places and 598 h 2e6 -generated hiding places. Seven seeking trials (5 for human hiding locations and 2 for artificial hiding locations) were not included due to participant error.
Static Image Representation Experiments
In these experiments we consider SIRs produced by our PVCNN when the PVCNN is trained using cache (taken from our final agent trained for 2,355,000 full episodes of cache), using an autoencoding loss (i.e. pixel-wise mean absolute error) with 35,855 AI2-THOR images, and with an image classification loss on the 1.28 million hand-labeled images in ImageNet [27] . SIR evaluation data and tasks To evaluate the quality of our SIRs we train decoder models on these SIRs to complete a number of distinct tasks described below. Except for in the classification task, where the decoder model is a max-pooling layer followed by a linear layer, the decoder models are designed similarly to those from a U-Net [42] , for details please see our open source code base. Depth prediction. In AI2-THOR we generate a collection of 3 × 224 × 224 RGB images paired with their corresponding 224 × 224 depth maps. Models are trained to predict the depth map given its corresponding RGB image by minimizing a per-pixel mean absolute error loss. Surface normals. In AI2-THOR we generate a collection of 3×224×224 RGB images where each image is paired with a corresponding 3 × 224 × 224 tensor T where T :,i,j represents the surface normal at pixel i, j in the RGB image. Models are trained to predict this tensor of surface normals given its corresponding RGB image by minimizing a per-pixel negative cosine similarity loss. Classification. In order to generate a dataset for classification we first select 90 types of objects available in AI2-THOR and assign each of these types a unique index in 1, ..., 90. Next, in AI2-THOR, we generate a collection of 3 × 224 × 224 RGB images where each image is paired with a {0, 1}-valued vector of length 90 whose jth entry equals 1 if and only if an object whose type has index j is present in the image and whose pixels occupy at least 1% of the image. Models are trained to predict this vector of 0,1 values from the input image using binary cross entropy. Openability. In AI2-THOR we generate a collection of 3 × 224 × 224 RGB images where each image is paired with a a 224 × 224 {0, 1, 2}-valued tensor T with entry T i,j equaling 1 if pixel i, j in the input image corresponds to an object that can be opened, equalling 2 if the pixel corresponds to an object that can be closed, and otherwise equals 0. Models are trained to predict the {0, 1, 2}valued tensor from the corresponding RGB image by minimizing a per-pixel mean cross entropy loss where the loss associated with predicting a 0 has a weight of 0.2 and the other losses have a weight of 1.0. Traversable surfaces prediction. In AI2-THOR we generate a collection of 3 × 224 × 224 RGB images where each image is paired with a corresponding 224 × 224 {0, 1}-valued tensor T with T i,j equalling one if and only if the ijth pixel in the image corresponds to a surface onto which the agent can walk. Models are trained to predict the {0, 1}-valued tensor given its corresponding RGB image by minimizing a per-pixel binary cross entropy loss. Object depth. In AI2-THOR we generate a collection of 3 × 224 × 224 RGB images each where each image is associated with a collection of 224 × 224 {0, 1}-valued masks of, sufficiently large, objects in the image paired with depth maps corresponding to the image in which the masked object has been removed. Models are trained to predict, from an input RGB image and object mask, the depth map of the image where the object has been removed by minimizing a per-pixel weighted mean absolute error loss. In this loss, pixels corresponding to the masked object are weighted to contribute equally to those pixels where the object is not present. Object surface normals. Similar to object depth but predicting surface normals after an object has been removed. Training We train using an ADAM optimizer with (β 1 , β 2 ) = (0.99, 0.999), a weight decay of 2e-6, and a plateau-based learning rate scheduler beginning with a learning rate of 1e-2 and decaying by a factor of 10 with a patience of N patience , that is, the scheduler reduces when the loss on the validation set has failed to decrease by some small absolute quantity for N patience subsequent epochs). When training the PCVNN to perform auto-encoding and ImageNet classification we used a patience of N patience = 5 and trained the models using all available training data. When training our other tasks for PVCNN, we used a patience of N patience = 5 and subsampled the training data to include 1,200 examples. Training was stopped if the learning rate ever decreased below 1e-4. During training models were evaluated on the validation set after every epoch and, once training completed, the model with the best validation loss was returned as the final model. Evaluation To evaluate our models we compute the loss of each model on each of the test set examples for its respective task. As any test set results generated within the same scene and at nearby location tuples are correlated, we use a generalized linear mixed effects model (GLMM) to obtain statistically meaningful comparisons between our models. We run our analysis using the R [38] programming language, in particular we use the glmmPQL function in the nlme [37] package to fit our GLMM models and then the emmeans [28] package to obtain p-values of contrasts between fixed effects. In these analyses we:
• Let the per test set example loss be our endogenous variable.
• Estimate fixed effects corresponding to which SIR was used to produce the loss (autoencoder, ImageNet, or cache).
• Include mixed effects corresponding to scene nested within scene type.
• Model spatial correlation, nested within scene, using spherical correlation where two test set examples corresponding to different SIRs or with different rotations are considered infinitely distant.
• Use a Gamma family model with an identity link.
• Subsample the test set to include at most 50 datapoints per scene and SIR so that the model can be more quickly trained.
To fully reproduce our results please see our open source code base.
Dynamic Image Representation Experiments
In all of our dynamic image representation experiments our DIRs are (some transformation of) the hidden state h s,t produced by the grid long short-term memory unit as described in the "information flow to policy and value" subsection for some stage s ∈ S stages and time t > 0. When this DIR needs to be collapsed to a single vector, we use 2d sum-pooling uponh s,t W s,t to obtain the 256 dimensional vector v s,t . Unless otherwise specified, SIRs correspond to the final output of the PVCNN after performing average pooling along the spatial dimensions so that the SIRs' dimensionality is 128. In the following we describe our training procedure for our dynamic image experiments along with any task-specific details including definitions of labels in the legend of Fig. 3 . Two such plot labels occur in three of our four experiments and so we describe them now. First DIR. (Rand.), corresponds to a randomly initialized agent with the same architecture as our cache agent and, in the below experiments, we will always use the same stage s and time t with this random agent as for our cache agent when computing its DIR. Secondly, DIR. (Nav.) corresponds to a navigation agent trained to perform the seeking task with object locations chosen from the sets of hiding locations, of easy and medium difficulty, generated by our exhaustive procedure. This navigation agent was trained, without VDR, for 150,000 episodes at which point training appeared to saturate. For tractability of inference, we will always consider our training set D train to be fixed and ignore any randomness, if applicable, in the generation of D train . Each of our considered tasks is a discrimination task and requires predicting, from a given representation, whether or not some binary condition holds. We call cases where the condition holds positive examples and others negative examples. We will accomplish these predictions by fitting a logistic regression models, details given in the training section below.
Training We wish to evaluate how our considered DI-and SI-representations perform in our tasks as the amount of data available for training varies when using a training procedure that follows best practices in machine learning by using dimensionality reduction and cross validation. Our procedure, based on iteratively subsampling D train , follows. Suppose we are interested in our representations' performance given a training set of size n > 0 with n < |D train |. We then repeat the following R > 0 times.
1. Subsample a training set D n ⊂ D train of size n taking care to retain as close to the same proportion of negative and positive examples as possible.
2. Perform dimensionality reduction on D n using (a variant of) principal component analysis, reducing the datasets dimensionality so that at least 99.99% of variance is explained by the remaining components, to obtain a datasetD n .
3. Fit a logistic regression model onD n using cross validation to choose an appropriate weighting of 2 -regularization. 4 . Apply the principal component analysis transformation learned in the second step to the test set D test to obtain aD test and then compute the test-accuracy on this transformed dataset.
This gives us R accuracy values a n1 , ..., a nR from which we can estimate the accuracy of our model on test examples when training our model on a randomly sampled training set of size n as the mean
a n = 1 R R i=1 a ni .
Note that randomness in the estimator a n comes both from our resampling procedure and, assuming our test set is not exhaustive, from the randomness in which examples where chosen to be included in the test set. The first source of randomness can be reduced simply by increasing R while the second can only be reduced by gather more test set examples. When appropriate, we account for both sources of randomness when plotting confidence intervals. Contained or behind relationships For these experiments we generate a dataset using the first five scenes of the foyer type in AI2-THOR. For each of these five scenes we generate a large collection of paired examples of objects being places into, or behind, one five receptacle objects while that receptacle sits on one of five possible tables at varying offsets from the agent. The placed objects are the same as those hidden in cache and we use five different receptacle objects of varying size. In order to make our setting especially difficult, we modify the above training procedure so that, in 1, we restrict our subsampled training set to exclude a randomly selected combination of scene, object, receptacle, and table. In 4 we then take our testing set to be the set of all examples in which the scene, object, receptacle, and table are exactly those excluded from the training set. This makes the test examples especially challenging as they are, visually, very dissimilar from those see during training. In this setting, only consider randomness as a result of our resampling procedure in our error estimates and so our inference is restricted to within our generated set of examples. Here our cache DIR is taken to be v OM,t from the OM-stage with goal location chosen to be the ONTOP modality with grid location i, j chosen to be overlaying receptacle object. Here t is chosen to be the time corresponding to the last action taken by the agent which leaves the object in its final position. Note that, unlike the cache agent, the navigation agent has no actions corresponding to hand movement and thus cannot, itself, move the object to the instructed location. In these cases we require the navigation agent to take a Stand agent at every time-step and move the object along the trajectory manually. Two baselines included in our results beyond the standard set of DIRs and SIRs are labeled as Forward Steps and SIR+ (ImageNet). Forward steps is simply the count of the number of MoveHandAhead actions taken as the object is moved along its trajectory to its final position. This baseline helps capture the significance of the bias that, in general, fewer MoveHandAhead actions are required when placing an object to be contained in, as opposed to behind, a receptacle. SIR+ (ImageNet), on the other hand, corresponds to a representation formed by concatenating together the standard ImageNet SIR (corresponding to the last frame), the ImageNet SIR generated from an image with the object removed, and the number of forward steps. Occluded object tracking As in our contained or behind relationship experiments we generate a dataset using the first five scenes of the foyer type in AI2-THOR and five table types. Here, as we are interested in tracking completely occluded objects, we use only three of our five item items, the cup, knife, and tomato, as these are sufficiently small to be frequently occluded. For each configuration of object, table, and scene, we generate a collection of object trajectories as the object moves behind a collection of books, recall Fig. 3b . For additional variability we produce many such trajectories with the books positioned in different distances and orientations. At every step of a trajectory where the object is occluded such that less than 10 pixels of the image correspond to the object we generate 49 data points, one for each grid location C ij on the 7 × 7 partition of the image, with the grid location being considered a positive example if, after making all other objects invisible, the primary object has greater than 10 pixels in that grid location and otherwise being consider negative. Note that, as objects tend to occupy only a small portion of an image, there are many more negative examples in our dataset than positive ones. During training we al-ways subsample negative examples so that there is an, approximately, equal number of positives and negatives. Furthermore, during testing, we implicitly balance positive negative examples by reweighting; thus the accuracy we report in this setting is a balanced accuracy. As in the previous experimental design, we modify the above training procedure so that, in 1, we restrict our subsampled training set to exclude a randomly selected combination of scene, object, and table. In 4 our testing set is those examples with exactly the configuration excluded from training. Again as above, only consider randomness as a result of our resampling procedure in our error estimates and so our inference is restricted to within our generated set of examples. Suppose that, at time t > 0, the object is fully occluded and X is a 7 × 7 {0, 1}-valued matrix with X ij equalling one only if grid position C ij is considered a positive example. Here we let the cache DIR for the grid position i, j in this case equal the 257-dimensional vector h OM,t :,i,j . The navigation DIR is generated using the same procedure. For our SIRs we also index into the 128 × 7 × 7 tensor T returned by the PVCNN to obtain the 128-dimensional vector T :,i,j . Similarly as in the previous experiment the navigation agent cannot move the object itself and so simply takes a Stand action at every timestep as the object is being moved. Object permanence when rotating In these experiments we first generate a large collection 3,594 (2,331 training, 646 validation, 614 testing) hiding locations from our h 2e6 agent. For each of these hiding locations we let our seeking agent attempt to find the object for 500 steps. If during one of these episodes, the agent would successfully take a ClaimVisible action at time t, so that it has found the object, we consider the counterfactual setting in which the agent instead rotates twice 90 • in the right or left direction. After each rotation we save the two DIRs v S,t and v S,t+1 as positive examples. As we are attempting to test our agents ability to remember the existence of an object once it is no longer in view, we ensure that the object is no longer visible after the first rotation by making it invisible. To create negative examples we replay this same episode, forcing the seeking agent to take all of the same actions, but with the goal object invisible during the entire trajectory. From this replayed trajectory we again save the two DIRs v S,t and v S,t+1 but assign them negative labels. Free space seriation In our final experiment we test the ability of our DIRs and SIRs to predict free space. We construct our dataset for this task by, for every AI2-THOR scene s, performing the following procedure. a. Move the agent to (x i , z i ), with a random axis aligned rotation, and randomly select a direction in which to rotate (left or right). The first set of rotations is a habituation phase.
b. Suppose we have chosen to rotate right. Then require the agent to take seven RotateRight actions so that every orientation is seen exactly twice. c. Save the DIRs, and SIRs, corresponding to the agents evaluation when looking in each orientation for the second time along with whether or not the current orientation has strictly more free space than the previous orientation. Free space is measured by the count of the grid locations that the agent could potentially occupy within the agent's visibility cone. Cases where a given orientation has strictly more free space are considered positive examples, the others negative.
3. Combine all positive and negative examples into our training and test datasets.
For this experiment our cache DIRs are generated with the agent as though it was taking actions in the E&M-stage, that is, from v E&M,t and similarly for the navigation agent.
Success Condition
Impact upon success Ep.
Moveahead, Moveleft, Moveright
No obstructions 0.25m in the direction of movement.
Agent moves 0.25m forward, left, or right respectively.
Rotateleft, Rotateright
If the agent is holding an object, it will not collide after rotation.
The agent rotates 90 • in the specified direction.
Stand, Crouch
Agent must begin crouching if taking a Stand action and must begin standing if taking a Crouch action. Additionally, if the agent is holding an object, this object will not collide with anything when the agent changes height.
Agent stands or crouches.
E&M, Oh, S
MoveHandAhead, MoveHandLeft, MoveHandRight, MoveHandBack, MoveHandUp, MoveHandDown
Agent must be holding an object, if so the agent applies a constant force on the object in the desired direction until it reaches a max velocity of 1m/s. The agent stops the object if it either moves a total of 0.1m from its starting position, the object reaches a max distance (1.5m) from the agent, the object exits the agent's viewport, or four seconds have elapsed. Once the agent stops the object, if the object has not moved more 0.001m or rotated at least 0.001 • then the movement is considered failed and the object is reset to its starting position. Otherwise hand movement was successful.
The hand object remains in its new position.
Dropobject
The agent is holding an object. The object is dropped.
Om
OpenAt|i,j, 1 ≤ i, j ≤ 7
Recall the partitioning of an image into a 7 × 7 grid as shown in Fig. 1d . There is an openable object near the center of the i, jth grid location.
The object near the location specified by i, j opens.
E&M, Oh, Om, S Closeobjects
There An object manipulation episode begins with target specified by i, j, m.
Oh
ReadyForSeeker The agent has successfully performed a PlaceAt action.
The hiding episode ends and the seeking episode begins.
Claimvisible
The goal object is within 1.5m of the agent and at least 10 pixels the agents current view can be attributed to the object.
The seeking episode ends successfully. S Table 1 : A description of the actions available to our agents along their success criteria, impact on the environment, and episodes in which they are available.
Algorithm 3: Object manipulation episode reward structure Input: Time step t ≥ 0, full history of OM episode H, number of steps taken in episode N , the target location specified by the action PlaceAt|m,i,j recall Table 1 Output: Reward for OM episode step at time t.