A Cordial Sync: Going Beyond Marginal Policies for Multi-Agent Embodied Tasks
Authors
Abstract
Autonomous agents must learn to collaborate. It is not scalable to develop a new centralized agent every time a task's difficulty outpaces a single agent's abilities. While multi-agent collaboration research has flourished in gridworld-like environments, relatively little work has considered visually rich domains. Addressing this, we introduce the novel task FurnMove in which agents work together to move a piece of furniture through a living room to a goal. Unlike existing tasks, FurnMove requires agents to coordinate at every timestep. We identify two challenges when training agents to complete FurnMove: existing decentralized action sampling procedures do not permit expressive joint action policies and, in tasks requiring close coordination, the number of failed actions dominates successful actions. To confront these challenges we introduce SYNC-policies (synchronize your actions coherently) and CORDIAL (coordination loss). Using SYNC-policies and CORDIAL, our agents achieve a 58% completion rate on FurnMove, an impressive absolute gain of 25 percentage points over competitive decentralized baselines. Our dataset, code, and pretrained models are available at this https URL .
1 Introduction
Collaboration is the defining principle of our society. Humans have refined strategies to efficiently collaborate, developing verbal, deictic, and kinesthetic means. In contrast, progress towards enabling artificial embodied agents to learn collaborative strategies is still in its infancy. Prior work mostly studies collaborative agents in grid-world like environments. Visual, multi-agent, collaborative tasks have not been studied until very recently [23, 42] . While existing tasks are well designed to study some aspects of collaboration, they often don't require agents to closely collaborate throughout the task. Instead such tasks either require initial coordination (distributing tasks) followed by almost independent execution, To study our algorithmic ability to address tasks which require close and frequent collaboration, we introduce the furniture moving (FurnMove) task (see Fig. 1 ), set in the AI2-THOR environment. Given only their egocentric visual observations, agents jointly hold a lifted piece of furniture in a living room scene and must collaborate to move it to a visually distinct goal location. As a piece of furniture cannot be moved without both agents agreeing on the direction, agents must explicitly coordinate at every timestep. Beyond coordinating actions, high performance in our task requires agents to visually anticipate possible collisions, handle occlusion due to obstacles and other agents, and estimate free space. Akin to the challenges faced by a group of roommates relocating a widescreen television, this task necessitates extensive and ongoing coordination amongst all agents at every time step.
In prior work, collaboration between multiple agents has been enabled primarily by (i) sharing observations or (ii) learning low-bandwidth communication. (i) is often implemented using a centralized agent, i.e., a single agent with access to all observations from all agents [9, 71, 93] . While effective it is also unrealistic: the real world poses restrictions on communication bandwidth, latency, and modality. We are interested in the more realistic decentralized setting enabled via option (ii). This is often implemented by one or more rounds of message passing between agents before they choose their actions [27, 58, 42] . Training decentralized agents when faced with FurnMove's requirement of coordination at each timestep leads to two technical challenges. Challenge 1: as each agent independently samples an action from its policy at every timestep, the joint probability tensor of all agents' actions at any given time is rank-one. This severely limits which multi-agent policies are representable. Challenge 2: the number of possible mis-steps or failed actions increases dramatically when requiring that agents closely coordinate with each other, complicating training.
Addressing challenge 1, we introduce SYNC (Synchronize Your actioNs Coherently) policies which permit expressive (i.e., beyond rank-one) joint policies for decentralized agents while using interpretable communication. To ameliorate challenge 2 we introduce the Coordination Loss (CORDIAL) that replaces the standard entropy loss in actor-critic algorithms and guides agents away from actions that are mutually incompatible. A 2-agent system using SYNC and CORDIAL obtains a 58% success rate on test scenes in FurnMove, an impressive absolute gain of 25 percentage points over the baseline from [42] (76% relative gain). In a 3-agent setting, this difference is even more extreme.
In summary, our contributions are: (i) FurnMove, a new multi-agent embodied task that demands ongoing coordination, (ii) SYNC, a collaborative mechanism that permits expressive joint action policies for decentralized agents, (iii) CORDIAL, a training loss for multi-agent setups which, when combined with SYNC, leads to large gains, and (iv) improvements to the open-source AI2-THOR environment including a 16× faster gridworld equivalent enabling fast prototyping.
2 Related Work
We start by reviewing single agent embodied AI tasks followed by non-visual Multi-Agent RL (MARL) and end with visual MARL. Single-agent embodied systems: Single-agent embodied systems have been considered extensively in the literature. For instance, literature on visual navigation, i.e., locating an object of interest given only visual input, spans geometric and learning based methods. Geometric approaches have been proposed separately for mapping and planning phases of navigation. Methods entailing structure-from-motion and SLAM [91, 80, 25, 13, 72, 81] were used to build maps. Planning algorithms on existing maps [14, 46, 52] and combined mapping & planning [26, 50, 49, 30, 6] are other related research directions.
While these works propose geometric approaches, the task of navigation can be cast as a reinforcement learning (RL) problem, mapping pixels to policies in an end-to-end manner. RL approaches [68, 1, 20, 33, 44, 92, 62, 86] have been proposed to address navigation in synthetic layouts like mazes, arcade games and other visual environments [100, 8, 47, 54, 43, 84] . Navigation within photo-realistic environments [11, 79, 15, 48, 102, 5, 35, 101, 59 ] led development of embodied AI agents. The early work [107] addressed object navigation (find an object given an image) in AI2-THOR. Soon after, [35] showed how imitation learning permits agents to learn to build a map from which they navigate. Methods also investigate the utility of topological and latent memory maps [35, 78, 37, 99] , graph-based learning [99, 103] , meta-learning [98] , unimodal baselines [90] , 3D point clouds [97] , and effective exploration [95, 78, 16, 74] to improve embodied navigational agents. Embodied navigation also aids AI agents to develop behavior such as instruction following [38, 4, 82, 95, 3] , city navigation [18, 64, 63, 94] , question answering [21, 22, 34, 97, 24] , and active visual recognition [105, 104] . Recently, with visual and acoustic rendering, agents have been trained for audio-visual embodied navigation [19, 31] .
In contrast to the above single-agent embodied tasks and approaches, we focus on collaboration between multiple embodied agents. Porting the above single-agent architectural novelties (or a combination of them) to multi-agent systems such as ours is an interesting direction for future work. Non-visual MARL: Multi-agent reinforcement learning (MARL) is challenging due to non-stationarity when learning. Multiple methods have been proposed to address resulting issues [88, 89, 87, 29] . For instance, permutation invariant critics have been developed recently [57] . In addition, for MARL, cooperation and competition between agents has been studied [51, 70, 60, 12, 69, 36, 58, 28, 57] . Similarly, communication and language in the multi-agent setting has been investigated [32, 45, 10, 61, 53, 27, 83, 67, 7] in maze-based setups, tabular tasks, or Markov games. These algorithms mostly operate on low-dimensional observations such as kinematic measurements (position, velocity, etc.) and top-down occupancy grids. For a survey of centralized and decentralized MARL methods, kindly refer to [106] . Our work differs from the aforementioned MARL works in that we consider complex visual environments. Our contribution of SYNC-Policies is largely orthogonal to RL loss function or method. For a fair comparison to [42] , we used the same RL algorithm (A3C) but it is straightforward to integrate SYNC into other MARL methods [75, 28, 58] (for details, see Sec. A.3 of the supplement). Visual MARL: Recently, Jain et al . [42] introduced a collaborative task for two embodied visual agents, which we refer as FurnLift. In this task, two agents are randomly initialized in an AI2-THOR living room scene, must visually navigate to a TV, and, in a singe coordinated PickUp action, work to lift that TV up. Note that FurnLift doesn't demand that agents coordinate their actions at each timestep. Instead, such coordination only occurs at the last timestep of an episode. Moreover, as success of an action executed by an agent is independent (with the exception of the PickUp action), a high performance joint policy need not be complex, i.e., it may be near low-rank. More details on this analysis and the complexity of our proposed FurnMove task are provided in Sec. 3 .
Similarly, a recent preprint [17] proposes a visual hide-and-seek task, where agents can move independently. Das et al . [23] enable agents to learn who to communicate with, on predominantly 2D tasks. In visual environments they study the task where multiple agents parallely navigate to the same object. Jaderberg et al . [41] recently studied the game of Quake III and Weihs et al . [96] develop agents to play an adversarial hiding game in AI2-THOR. Collaborative perception for semantic segmentation and recognition classification have also been investigated recently [55, 56] .
To the best of our knowledge, all previous visual or non-visual MARL in the decentralized setting operate with a single marginal probability distribution per agent, i.e., a rank-one joint distribution. Moreover, FurnMove is the first multiagent collaborative task in a visually rich domain requiring close coordination between agents at every timestep.
3 The Furniture Moving Task (Furnmove)
We describe our new multi-agent task FurnMove, grounded in the real-world experience of moving furniture. We begin by introducing notation. RL background and notation. Consider N ≥ 1 collaborative agents A 1 , . . . , A N . At every timestep t ∈ N = {0, 1, . . .} the agents, and environment, are in some state s t ∈ S and each agent A i obtains an observation o i t recording some partial information about s t . For instance, o i t might be the egocentric visual view of an agent A i embedded in some simulated environment. From observation o i t and history h i t−1 , which records prior observations and decisions made by the agent, each agent A i forms a policy π i t : A → [0, 1] where π i t (a) is the probability that agent A i chooses to take action a ∈ A from a finite set of options A at time t. After the agents execute their respective actions (a 1 t , . . . , a N t ), which we call a multi-action, they enter a new state s t+1 and receive individual rewards
r 1 t , . . . , r N t ∈ R.
For more on RL see [85, 65, 66] . Task definition. FurnMove is set in the near-photorealistic and physics enabled simulated environment AI2-THOR [48] . In FurnMove, N agents collaborate to move a lifted object through an indoor environment with the goal of placing this object above a visually distinct target as illustrated in Fig. 1 . Akin to humans moving large items, agents must navigate around other furniture and frequently walk in-between obstacles on the floor.
In FurnMove, each agent at every timestep receives an egocentric observation (a 3 × 84 × 84 RGB image) from AI2-THOR. In addition, agents are allowed to communicate with other agents at each timestep via a low bandwidth communication channel. Based on their local observation and communication, each agent must take an action from the set A. The space of actions A = A NAV ∪ A MWO ∪ A MO ∪ A RO available to an agent is comprised of the four single-agent navigational actions A NAV = {MoveAhead, RotateLeft, Rota-teRight, Pass} used to move the agent independently, four actions A MWO = {MoveWithObjectX | X ∈ {Ahead, Right, Left, Back}} used to move the lifted object and the agents simultaneously in the same direction, four actions A MO = {MoveObjectX| X ∈ {Ahead, Right, Left, Back}} used to move the lifted object while the agents stay in place, and a single action used to rotate the lifted object clockwise A RO = {RotateObjectRight}. We assume that all movement actions for agents and the lifted object result in a displacement of 0.25 meters (similar to [42, 59] ) and all rotation actions result in a rotation of 90 degrees (counter-)clockwise when viewing the agents from above. Close and on-going collaboration is required in FurnMove due to restrictions on the set of actions which can be successfully completed jointly by all the agents. These restrictions reflect physical constraints: for instance, if two people attempt to move in opposite directions while carrying a heavy object they will either fail to move or drop the object. For two agents, we summarize these restrictions using the coordination matrix shown in Fig. 2a . For comparison, we include a similar matrix in Fig. 2b corresponding to the FurnLift task from [42] . We defer a more detailed discussion of these restrictions to Sec. A.1 of the supplement. Generalizing the coordination matrix shown in Fig. 2a , at every timestep t we let S t be the {0, 1}-valued |A| N -dimensional tensor where (S t ) i1,...,i N = 1 if and only if the agents are configured such that multi-action (a i1 , . . . , a i N ) satisfies the restrictions detailed in Sec. A.1. If (S t ) i1,...,i N = 1 we say the actions (a i1 , . . . , a i N ) are coordinated.
X X X X X X X X X X X X X X X X X X X X X X X X X X X X X X X X X X X X X X X X X X X X X X X X X X X X X X X X X X X X X X X X X X X X X X X X X X X X X X X X X X X X X X X X X X X X X X X X X X X X X X X X X X X X X X X X X X X X X X X X X X X X X X X X X X X X X X X X X X X X X X X X X X X X X X X X X X X X X X X X X X X X X X X X X MAhead
3.1 Technical Challenges
As we show in our experiments in Sec. 6, standard communication-based models similar to the ones proposed in [42] perform rather poorly when trained to complete the FurnMove task. In the following we identify two key challenges that contribute to this poor performance. Challenge 1: rank-one joint policies. In classical multi-agent settings [12, 70, 58] [42] backbone architecture. Right: marginal vs SYNC-policies. With marginal policies, the standard in prior work, each agent constructs its own policy and independently samples from this policy. With SYNC-policies, agents communicate to construct a distribution α over multiple "strategies" which they then sample from using a shared random seed to this independent sampling, at time t, the probability of the agents taking multi
-action (a 1 , . . . , a N ) equals N i=1 π i t (a i )
. This means that the joint probability tensor of all actions at time t can be written as the rank-one tensor
Π t = π 1 t ⊗ • • • ⊗ π N t .
This rank-one constraint limits the joint policy that can be executed by the agents, which has real impact. Sec. A.2 considers two agents playing rock-paper-scissors with an adversary: the rank-one constraint reduces the expected reward achieved by an optimal policy from 0 to -0.657 (minimal reward being -1). Intuitively, a high-rank joint policy is not well approximated by a rank-one probability tensor obtained via independent sampling. Challenge 2: exponential failed actions. The number of possible multiactions |A| N increases exponentially as the number of agents N grows. While this is not problematic if agents act relatively independently, it's a significant obstacle when the agents are tightly coupled, i.e., when the success of agent A i 's action a i is highly dependent on the actions of the other agents. Just consider a randomly initialized policy (the starting point of almost all RL problems): agents stumble upon positive rewards with an extremely low probability which leads to slow learning. We focus on small N , nonetheless, the proportion of coordinated action tuples is small (9.5% when N = 2 and 2.1% when N = 3).
4 A Cordial Sync
To address the aforementioned two challenges we develop: (a) a novel action sampling procedure named Synchronize Your actioNs Coherently (SYNC) and (b) an intuitive & effective multi-agent training loss named the Coordination Loss (CORDIAL). Addressing challenge 1: SYNC-policies. For readability, we consider N = 2 agents and illustrates an overview in Fig. 3 . The joint probability tensor Π t is hence a matrix of size |A| × |A|. Recall our goal: using little communication, multiple agents should sample their actions from a high-rank joint policy. This is difficult as (i) little communication means that, except in degenerate cases, no agent can form the full joint policy and (ii) even if all agents had access to the joint policy it is not obvious how to ensure that the decentralized agents will sample a valid coordinated action.
To achieve this note that, for any rank m ≤ |A| matrix L ∈ R |A|×|A| , there are
vectors v 1 , w 1 , . . . , v m , w m ∈ R |A| such that L = m j=1 v j ⊗ w j .
Here, ⊗ denotes the outer product. Also, the non-negative rank of a matrix L ∈ R |A|×|A| ≥0 equals the smallest integer s such that L can be written as the sum of s non-negative rank-one matrices. Furthermore, a non-negative matrix L ∈ R
|A|×|A| ≥0
has nonnegative rank bounded above by |A|. Since Π t is a |A|×|A| joint probability matrix, i.e., Π t is non-negative and its entries sum to one, it has non-negative rank m ≤ |A|, i.e., there exist non-negative vectors α ∈ R m
≥0 and p 1 , q 1 , . . . , p m , q m ∈ R |A| ≥0 whose entries sum to one such that Π t = m j=1 α j • p j ⊗ q j .
We call a sum of the form
m j=1 α j • p j ⊗ q j a mixture-of-marginals. With this decomposition at hand, randomly sampling action pairs (a 1 , a 2 ) from m j=1 α j • p j ⊗ q j
can be interpreted as a two step process: first sample an index j ∼ Multinomial(α) and then sample a 1 ∼ Multinomial(p j ) and a 2 ∼ Multinomial(q j ).
This stage-wise procedure suggests a strategy for sampling actions in a multiagent setting, which we refer to as SYNC-policies. Generalizing to an N agent setup, suppose that agents (A i ) N
i=1 have access to a shared random stream of numbers. This can be accomplished if all agents share a random seed or if all agents initially communicate their individual random seeds and sum them to obtain a shared seed. Furthermore, suppose that all agents locally store a shared function f θ : R K → ∆ m−1 where θ are learnable parameters, K is the dimensionality of all communication between the agents in a timestep, and ∆ m−1 is the standard (m − 1)-probability simplex. Finally, at time t suppose that each agent A i produces not a single policy π i t but instead a collection of policies π i t,1 , . . . , π i t,m . Let C t ∈ R K be all communication sent between agents at time t. Each agent A i then samples its action as follows: (i) compute the shared probabilities α t = f θ (C t ), (ii) sample an index j ∼ Multinomial(α t ) using the shared random number stream, (iii) sample, independently, an action a i from the policy π i t,j . Since both f θ and the random number stream are shared, the quantities in (i) and (ii) are equal across all agents despite being computed individually. This sampling procedure is equivalent to sampling from the tensor
m j=1 α j • π 1 t,j ⊗ . . . ⊗ π N t,j
which, as discussed above, may have rank up to m. Intuitively, SYNC enables decentralized agents to have a more expressive joint policy by allowing them to agree upon a strategy by sampling from α t . Addressing challenge 2: CORDIAL. We encourage agents to rapidly learn to choose coordinated actions via a new loss. In particular, letting Π t be the joint policy of our agents, we propose the coordination loss (CORDIAL)
EQUATION (1): Not extracted; please refer to original document.
where log is applied element-wise, * , * is the usual Frobenius inner product, and S t is defined in Sec. 3. Notice that CORDIAL encourages agents to have a near uniform policy over the actions which are coordinated. We use this loss to replace the standard entropy encouraging loss in policy gradient algorithms (e.g., the A3C algorithm [66] ). Similarly to the parameter for the entropy loss in A3C, β is chosen to be a small positive constant so as to not overly discourage learning.
Note that the coordination loss is less meaningful when
Π t = π 1 ⊗ • • • ⊗ π N , i.e.
, when Π t is rank-one. For instance, suppose that S t has ones along the diagonal, and zeros elsewhere, so that we wish to encourage the agents to all take the same action. In this case it is straightforward to show that
CL β (S t , Π t ) = −β N i=1 M j=1 (1/M ) log π i
t (a j ) so that CL β (S t , Π t ) simply encourages each agent to have a uniform distribution over its actions and thus actually encourages the agents to place a large amount of probability mass on uncoordinated actions. Indeed, Tab. 4 shows that using CORDIAL without SYNC leads to poor results.
5 Models
We study four distinct policy types: central, marginal, marginal w/o comm, and SYNC . Central samples actions from a joint policy generated by a central agent with access to observations from all agents. While often unrealistic in practice due to communication bottlenecks, central serves as an informative baseline. Marginal follows prior work, e.g., [42] : each agent independently samples its actions from its individual policy after communication. Marginal w/o comm is identical to marginal but does not permit agents to communicate explicitly (agents may still see each other). Finally, SYNC is our newly proposed policy described in Sec. 4. For a fair comparison, all decentralized agents (i.e., SYNC , marginal, and marginal w/o comm), use the same TBONE backbone architecture from [42] , see Fig. 3 . We have ensured that parameters are fairly balanced so that our proposed SYNC has close to (and never more) parameters than the marginal and marginal w/o comm nets. Note, we train central and SYNC with CORDIAL, and the marginal and marginal w/o comm without it. This choice is mathematically explained in Sec. 4 and empirically validated in Sec. 6.3. Architecture details: For readability we describe the policy and value net for the 2 agent setup while noting that it can be trivially extended to any number of agents. As noted above, decentralized agents use the TBONE backbone from [42] . Our primary architectural novelty extends TBONE to SYNC-policies. An overview of the TBONE backbone and differences between sampling with marginal and SYNC policies is shown in Fig. 3 .
As a brief summary of TBONE, agent i observes at time t inputs o i t , i.e., a 3×84×84 RGB image returned from AI2-THOR which represents the i-th agent's egocentric view. For each agent, the observation is encoded by a four layer CNN and combined with an agent specific learned embedding (that encodes the ID of that agent) along with the history embedding h i t−1 . The resulting vector is fed into a long-short-term-memory (LSTM) [39] unit to produce a 512-dimensional embeddingh i t corresponding to the i th agent. The agents then undergo two rounds of communication resulting in two final hidden states h 1 t , h 2 t and messages
c i t,j ∈ R 16 , 1 ≤ i, j ≤ 2 with message c i t,j
being produced by agent i in round j and then sent to the other agent in that round. In [42] , the value of the agents' state as well as logits corresponding to the policy of the agents are formed by applying linear functions to h 1 t , h 2 t . We now show how SYNC can be integrated into TBONE to allow our agents to represent high rank joint distributions over multi-actions (see Fig. 3 ). First each agent computes the logits corresponding to α t . This is done using a 2-layer MLP applied to the messages sent between the agents, at the second stage. In particular, 64 , and b 3 ∈ R m are a learnable collection of weight matrices and biases. After computing α t we compute a collection of policies π i t,1 , . . . , π i t,m for i ∈ {1, 2}. Each of these policies is computed following the TBONE architecture but using m − 1 additional, and learnable, linear layers per agent.
α t = W 3 ReLU(W 2 ReLU(W 1 [c 1 t,2 ; c 2 t,2 ] + b 1 ) + b 2 ) + b 3 where W 1 ∈ R 64×32 , W 2 ∈ R 64×64 , W 3 ∈ R m×64 , b 1 ∈ R 32 , b 2 ∈ R
6.1 Experimental Setup
Simulator. We evaluate our models using the AI2-THOR environment [48] with several novel upgrades. First, we introduce new methods which permit to (a) randomly initialize the lifted object and agent locations close to each other and looking towards the lifted object, and (b) simultaneously move agents and the lifted object in a given direction with collision detection. Secondly, we build a top-down gridworld version of AI2-THOR for faster prototyping, that is 16× faster than [42] . For details about framework upgrades, see Sec. A.3 of the supplement. Tasks. We compare against baselines on FurnMove, Gridworld-FurnMove, and FurnLift [42] . FurnMove is the novel task introduced in this work (Sec. 3): agents observe egocentric visual views, with field-of-view 90 degrees. In FurnMove-Gridworld the agents are provided a top-down egocentric 3D tensor as observations. The third dimension of the tensor contains semantic information such as, if the location is navigable by an agent or navigable by the lifted object, or whether the location is occupied by another agent, the lifted object, or the goal object. Hence, FurnMove-Gridworld agents do not need visual understanding, but face other challenges of the FurnMove task -coordinating actions and planning trajectories. We consider only the harder variant of FurnLift, where communication was shown to be most important ('constrained' with no implicit communication in [42] ). In FurnLift, agents observe egocentric visual views. Data. As in [42] , we train and evaluate on a split of the 30 living room scenes. As FurnMove is already quite challenging, we only consider a single piece of lifted furniture (a television) and a single goal object (a TV-stand). Twenty rooms are used for training, 5 for validation, and 5 for testing. The test scenes have very different lighting conditions, furniture, and layouts. For evaluation our test set includes 1000 episodes equally distributed over the five scenes. Training. For training we augment the A3C algorithm [66] with CORDIAL. For our studies in the visual domain, we use 45 workers and 8 GPUs. Models take around two days to train. For more details about training, including hyperparameter values and the reward structure, see Sec. A.3 of the supplement.
6.2 Metrics
For completeness, we consider a variety of metrics. We adapt SPL, i.e., Success weighted by (normalized inverse) Path Length [2] , so that it doesn't require shortest paths but still provides similar semantic information 4 : We define a Manhattan Distance based SPL as MD
-SPL = N −1 ep Nep i=1 S i mi/d grid max(pi,mi/d grid )
, where i denotes an index over episodes, N ep equals the number of test episodes, and S i is a binary indicator for success of episode i. Also p i is the number of actions taken per agent, m i is the Manhattan distance from the lifted object's start location to the goal, and d grid is the distance between adjacent grid points, for us 0.25m. We also report other metrics capturing complementary information. These include mean number of actions in an episode per agent (Ep len), success rate (Success), and distance to target at the end of the episode (Final dist).
We also introduce two metrics unique to coordinating actions: TVD, the mean total variation distance between Π t and its best rank-one approximation, and Invalid prob, the average probability mass allotted to uncoordinated actions, i.e., the dot product between 1 − S t and Π t . By definition, TVD is zero for the marginal model, and higher values indicate divergence from independent marginal sampling. Note that, without measuring TVD we would have no way of knowing if our SYNC model was actually using the extra expressivity we've afforded it. Lower Invalid prob values imply an improved ability to avoid uncoordination actions as detailed in Sec. 3
6.3 Quantitative Evaluation
We conduct four studies: (a) performance of different methods and relative difficulty of the three tasks, (b) effect of number of components on SYNC performance, (c) effect of CORDIAL (ablation), and (d) effect of number of agents. Comparing methods and tasks. We compare models detailed in Sec. 5 on tasks of varying difficulty, report metrics in Tab. 1, and show the progress of metrics over training episodes in Fig. 4 . In our FurnMove experiments, SYNC performs better than the best performing method of [42] (i.e., marginal ) on all metrics. Success rate increases by 25.9% and 6.8% absolute percentage points on FurnMove and Gridworld-FurnMove respectively. Importantly, SYNC is significantly better at allowing agents to coordinate their actions: for FurnMove, the joint policy of SYNC assigns, on average, 0.31 probability mass to invalid actions pairs while the marginal and marginal w/o comm models assign 0.647 and 0.815 probability mass to invalid action pairs. Additionally, SYNC goes beyond rank-one marginal methods by capturing a more expressive joint policy using the mixture of marginals. This is evidenced by the high TVD of 0.474 vs. 0 for marginal. In Gridworld-FurnMove, oracle-perception of a 2D gridworld helps raise performance of all methods, though the trends are similar. Tab. 2 shows similar trends for FurnLift but, perhaps surprisingly, the Success of SYNC is somewhat lower than the marginal model (2.6% lower, within statistical error). As is emphasized in [42] however, Success alone is a poor measure of model performance: equally important are the failed pickups and missed pickups metrics (for details, see Sec. A.4 of the supplement). For these metrics, SYNC outperforms the marginal model. That SYNC does not completely outperform marginal in FurnLift is intuitive, as FurnLift does not require continuous close coordination the benefits of SYNC are less pronounced. While the difficulty of a task is hard to quantify, we will consider the relative test-set metrics of agents on various tasks as an informative proxy. Replacing the complex egocentric vision in FurnMove with the semantic 2D gridworld in Gridworld-FurnMove, we see that all agents show large gains in Success and MD-SPL, suggesting that Gridworld-FurnMove is a dramatic simplification of FurnMove. Comparing FurnMove to FurnLift is particularly interesting. The MD-SPL and Success metrics for the central agent do not provide a clear indication regarding task difficulty amongst the two. However, notice the much higher TVD for the central agent for FurnMove and the superior MD-SPL and Success of the Marginal agents for FurnLift. These numbers clearly indicate that FurnMove requires more coordination and additional expressivity of the joint distribution than FurnLift. Effect of number of mixture components in SYNC. Recall (Sec. 4) that the number of mixture components m in SYNC is a hyperparameter controlling the maximal rank of the joint policy. SYNC with m = 1 is equivalent to marginal. In Tab. 3 we see T V D increase from 0.206 to 0.474 when increasing m from 2 to 13. This suggests that SYNC learns to use the additional expressivity. Moreover, we see that this increased expressivity results in better performance. A success rate jump of 17.4% from m = 1 to m = 2 demonstrates that substantial benefits are obtained by even small increases in expressitivity. Moreover with more components, i.e., m = 4 & m = 13 we obtain more improvements. Notice Effect of more agents. The final three rows of Tab. 1 show the test-set performance of SYNC , marginal, and central models trained to accomplish a 3-agent variant of our Gridworld-FurnMove task. In this task the marginal model fails to train at all, achieving a 0% success rate. SYNC , on the other hand, successfully completes the task 57.8% of the time. Notice that SYNC 's success rate drops by nearly 20 percentage points when moving from the 2-to the 3-agent variant of the task: clearly increasing the number of agents substantially increases the task's difficult. Surprisingly, the central model performs worse than SYNC in this setting. A discussion of this phenomenon is deferred to Sec. A.4 of the supplement.
6.4 Qualitative Evaluation
We present three qualitative results on FurnMove: joint policy summaries, analysis of learnt communication, and visualizations of agent trajectories. Joint policy summaries. In Fig. 5 we show summaries of the joint policy captured by the central, SYNC , and marginal models. These matrices average over action steps in the test-set episodes for FurnMove. Other tasks show similar trends, see Sec. A.5 of the supplement. In Fig. 5a , the sub-matrices corresponding to A MWO and A MO are diagonal-dominant, indicating that agents are looking in the same direction (0 • relative orientation in Fig. 2) . Also note the high probability associated to (Pass, RotateX) and (RotateX, Pass), within the A NAV block. Together, this means that the central method learns to coordinate single-agent navigational actions to rotate one of the agents (while the other holds the TV by executing Pass) until both face the same direction. They then execute the same action from A MO (A MWO ) to move the lifted object. Comparing Fig. 5b vs. Fig. 5c , shows the effect of CORDIAL. Recall that the marginal model doesn't support CORDIAL and thus suffers by assigning probability to invalid action pairs (color outside the block-diagonal submatrices). Also note the banded nature of Fig. 5c resulting from its construction as an outer product of marginals. Communication analysis. A qualitative discussion of communication follows. Agent are colored red and green. We defer a quantitative treatment to Sec. A.5 of the supplement. As we apply SYNC on the TBONE backbone introduced by Jain et al . [42] , we use similar tools to understand the communication emerging with SYNC policy heads. In line with [42] , we plot the weight assigned by each agent to the first communication symbol in the reply stage. Fig. 5d strongly suggests that the reply stage is directly used by the agents to coordinate the modality of actions they intend to take. In particular, note that a large weight being assigned to the first reply symbol is consistently associated with the other agent taking a Pass action. Similarly, we see that small reply weights coincide with agents taking a MoveWithObject action. The talk weights' interpretation is intertwined with the reply weights, and is deferred to Sec. A.5 of the supplement. Agent trajectories. Our supplementary video includes examples of policy rollouts. These clips include both agents' egocentric views and a top-down trajectory visualization. This enables direct comparisons of marginal and SYNC on the same test episode. We also allow for hearing patterns in agents' communication: we convert scalar weights (associated with reply symbols) to audio.
7 Conclusion
We introduce FurnMove, a collaborative, visual, multi-agent task requiring close coordination between agents and develop novel methods that allow for moving beyond existing marginal action sampling procedures, these methods lead to large gains across a diverse suite of metrics.
A.1 Action Restrictions
We now comprehensively describe the restrictions defining when actions taken by agents are globally consistent with one another. In the following we will, for readability, focus on the two agent setting. All conditions defined here easily generalize to any number of agents. Recall the sets A NAV , A MWO , A MO , and A RO defined in Sec. 3. We call these sets the modalities of action. Two actions a 1 , a 2 ∈ A are said to be of the same modality if they both are an element of the same modality of action. Let a 1 and a 2 be the actions chosen by the two agents. Below we describe the conditions when a 1 and a 2 are considered coordinated. If the agents' actions are uncoordinated, both actions fail and no action is taken for time t. These conditions are summarized in Fig. 2a . Same action modality. A first necessary, but not sufficient, condition for successful coordination is that the agents agree on the modality of action to perform. Namely that both a 1 and a 2 are of the same action modality. Notice the block diagonal structure in Fig. 2a . No independent movement. Our second condition models the intuitive expectation that if one agent wishes to reposition itself by performing a singleagent navigational action, the other agent must remain stationary. Thus, if a 1 , a 2 ∈ A NAV , then (a 1 , a 2 ) are coordinated if and only if one of a 1 or a 2 is a Pass action. The {1, 2, 3, 4} 2 entries of the matrix in Fig. 2a show coordinated pairs of single-agent navigational actions. Orientation synchronized object movement. Suppose that both agents wish to move (with) the object in a direction so that a 1 , a 2 ∈ A MWO or a 1 , a 2 ∈ A MO . Note that, as actions are taken from an egocentric perspective, it is possible, for example, that moving ahead from one agent's perspective is the same as moving left from the other's. This condition requires that the direction specified by both of the agents is consistent globally. Hence a 1 , a 2 are coordinated if and only if the direction specified by both actions is the same in a global reference frame. For example, if both agents are facing the same direction this condition requires that a 1 = a 2 while if the second agent is rotated 90 degrees clockwise from the first agent then a 1 = MoveObjectAhead will be coordinated if and only if a 2 = MoveObjectLeft. See the multicolored 4×4 blocks in Fig. 2a . Simultaneous object rotation. For the lifted object to be rotated, both agents must rotate it in the same direction in a global reference frame. As we only allow the agents to rotate the object in a single direction (clockwise) this means that a 1 = RotateObjectRight requires a 2 = a 1 . See the (9, 9) entry of the matrix in Fig. 2a . While a pair of uncoordinated actions are always unsuccessful, it need not be true that a pair of coordinated actions is successful. A pair of coordinated actions will be unsuccessful in two cases: performing the action pair would result in (a) an agent, or the lifted object, colliding with one another or another object in the scene; or (b) an agent moving to a position more than 0.76m from the lifted object. Here (a) enforces the physical constraints of the environment while (b) makes the intuitive requirement that an agent has a finite reach and cannot lift an object when being far away.
A.2 Challenge 1 (Rank-One Joint Policies) Example
We now illustrate how requiring two agents to independently sample actions from marginal policies can result in failing to capture an optimal, high-rank, joint policy.
Consider two agents A 1 and A 2 who must work together to play rock-paperscissors (RPS) against some adversary E. In particular, our game takes place in a single timestep where each agent A i , after perhaps communicating with the other agent, must choose some action a i ∈ A = {R, P, S}. During this time the adversary also chooses some action a E ∈ A. Now, in our game, the pair of agents A 1 , A 2 lose if they choose different actions (i.e., a 1 = a 2 ), tie with the adversary if all players choose the same action (i.e., a 1 = a 2 = a E ), and finally win or lose if they jointly choose an action that beats or losses against the adversary's choice following the normal rules of RPS (i.e., win if (a 1 , a 2 , a E ) ∈ {(R, R, S), (P, P, R), (S, S, P )}, lose if (a 1 , a 2 , a E ) ∈ {(S, S, R), (R, R, P ), (P, P, S)}).
Moreover, we consider the challenging setting where A 1 , A 2 communicate in the open so that the adversary can view their joint policy Π before choosing the action it wishes to take. Notice that we've dropped the t subscript on Π as there is only a single timestep. Finally, we treat this game as zero-sum so that our agents obtain a reward of 1 for victory, 0 for a tie, and -1 for a loss. We refer to the optimal joint policy as Π * . If the agents operate in a decentralized manner using their own (single) marginal policies, their effective rank-one joint policy equals Π = π 1 ⊗ π 2 . Optimal joint policy: It is well known, and easy to show, that the optimal joint policy equals Π * = I 3 /3, where I 3 is the identity matrix of size 3. Hence, the agents take multi-action (R, R), (P, P ), or (S, S) with equal probability obtaining an expected reward of zero. Optimal rank-one joint policy: Π * (the optimal joint policy) is of rank three and thus cannot be captured by Π (an outer product of marginals). Instead, brute-force symbolic minimization, using Mathematica [40] , shows that an optimal strategy for A 1 and A 2 is to let
EQUATION (2): Not extracted; please refer to original document.
π 1 (P ) = 0, and (3)
EQUATION (4): Not extracted; please refer to original document.
The expected reward from this strategy is 5 − 4 √ 2 ≈ −.657, far less than the optimal expected reward of 0.
A.3 Training Details
Centralized agent. Fig. 6 provides an overview of the architecture of the centralized agent. The final joint policy is constructed using a single linear layer applied to a hidden state. As this architecture varies slightly when changing the number of agents and the environment (i.e., AI2-THOR or our gridworld variant of AI2-THOR) we direct anyone interested in exact replication to our codebase.
AI2-THOR upgrades. As we described in Sec. 6 we have made several upgrades to AI2-THOR in order to make it possible to run our FurnMove task. These upgrades are described below. Implementing FurnMove methods in AI2-THOR's Unity codebase. The AI2-THOR simulator has been built using C# in Unity. While multi-agent support exists in AI2-THOR, our FurnMove task required implementing a collection of new methods to support randomly initializing our task and moving agents in tandem with the lifted object. Initialization is accomplished by a randomized search procedure that first finds locations in which the lifted television can be placed and then determines if the agents can be situated around the lifted object so that they are sufficiently close to the lifted object and looking at it. Implementing the joint movement actions (recall A MWO ) required checking that all agents and objects can be moved along straight-line paths without encountering collisions. Top-down Gridworld Mirroring AI2-THOR. To enable fast prototyping and comparisons between differing input modalities, we built an efficient gridworld mirroring AI2-THOR. See Fig. 7 for a side-by-side comparison of AI2- THOR and our gridworld. This gridworld was implemented primarily in Python with careful caching of data returned from AI2-THOR.
Reward structure. Rewards are provided to each agent individually at every step. These rewards include: (a) +1 whenever the lifted object is moved closer, in Euclidean distance, to the goal object than it had been previously in the episode, (b) a constant −0.01 step penalty to encourage short trajectories, and (c) a penalty of −0.02 whenever the agents action fails. The minimum total reward achievable for a single agent is −7.5 corresponding to making only failed actions, while the maximum total reward equals 0.99 • d where d is the total number of steps it would take to move the lifted furniture directly to the goal avoiding all obstructions. Our models are trained to maximize the expected discounted cumulative gain with discounting factor γ = 0.99.
Optimization and learning hyperparameters. For all tasks, we train our agents using reinforcement learning, particularly the popular A3C algorithm [66] . For FurnLift, we follow [42] and additionally use a warm start via imitation learning (DAgger [77] ). When we deploy the coordination loss (CORDIAL), we modify the A3C algorithm by replacing the entropy loss with the coordination loss CORDIAL defined in Eq. (1) . In our experiments we anneal the β parameter from a starting value of β = 1 to a final value of β = 0.01 over the first 5000 episodes of training. We use an ADAM optimizer with a learning rate of 10 −4 , momentum parameters of 0.9 and 0.999, with optimizer statistics shared across processes. Gradient updates are performed in an unsynchronized fashion using a HogWild! style approach [76] . Each episode has a maximum length of 250 total steps per agent. Task-wise details follow:
-FurnMove: Visual agents for FurnMove are trained for 500, 000 episodes, across 8 TITAN V or TITAN X GPUs with 45 workers and take approximately three days to train. -Gridworld-FurnMove: Agents for Gridworld-FurnMove are trained for 1,000,000 episodes using 45 workers. Apart from parsing and caching the scene once, gridworld agents do not need to render images. Hence, we train the agents with only 1 G4 GPU, particularly the g4dn.16xlarge virtual machine on AWS. Agents (i.e., two) for Gridworld-FurnMove take approximately 1 day to train. -Gridworld-FurnMove-3Agents: Same implementation as above, except that agents (i.e., three) for Gridworld-FurnMove-3Agents take approximately 3 days to train. This is due to an increase in the number of forward and backward passes and a CPU bottleneck. Due to the action space blowing up to |A| × |A| × |A| = 2197 (vs. 169 for two agents), positive rewards become increasingly sparse. This leads to grave inefficiency in training, with no learning for ∼500k episodes. To overcome this, we double the positive rewards for the RL formulation for all methods within the three agent setup. -FurnLift: We adhere to the exact training procedure laid out by Jain et al . [42] . Visual agents for FurnLift are trained for 100,000 episodes with the first 10,000 being warm started with a DAgger-styled imitation learning. Reinforcement learning (A3C) takes over after the warm-start period.
Integration with other MARL methods. As mentioned in Sec. 2, our contributions are orthogonal to the RL method deployed. Here we give some pointers for integration with a deep Q-Learning and a policy gradient method. QMIX. While we focus on policy-gradients and QMIX [75] uses Q-learning, we can formulate a SYNC for Q-Learning (and QMIX). Analogous to an actor with multiple policies, consider a value head where each agent's Q-function Q i is replaced by a collection of Q-functions Q a i for a ∈ A. Action sampling is done stagewise, i.e. agents jointly pick a strategy as arg max a Q SY N C (communications, a), and then individually choose action arg max u i Q a i (τ i , u i ). These Q a i in turn can incorporated into the QMIX mixing network. COMA/MADDPG. Both these policy gradient algorithms utilize a centralized critic. Since our contributions focus on the actor head, we can directly replace their per-agent policy with our SYNC policies and thus benefit directly from the counterfactual baseline in COMA [28] or the centralized critic in MAD-DPG [58] .
A.4 Quantitative Evaluation Details
Confidence intervals for metrics reported. In the main paper, we mentioned that we mark the best performing decentralized method in bold and highlight it in green if it has non-overlapping 95% confidence intervals. In this supplement, particularly in Tab. 5, Tab. 6, Tab. 7, and Tab. 8 we include the 95% confidence intervals for the metrics reported in Tab. 1, Tab. 2, Tab. 3, and Tab. 4.
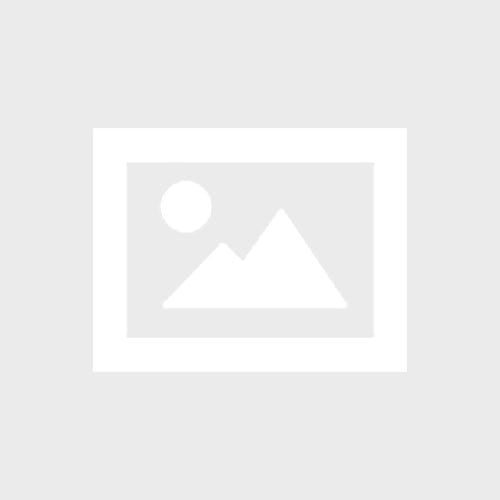
Hypotheses on 3-agent central method performance. In Fig. 1 and Sec. 6.3 of the main paper, we mention that the central method performs worse than SYNC for the Gridworld-FurnMove-3Agent task. We hypothesize that this is because the central method for the -3Agent setup is significantly slower as its actor head has dramatically more parameters requiring more time to train. In numbers -the central 's actor head alone has D × |A| 3 parameters, where D is the dimensionality of the final representation fed into the actor (please see Fig. 6 for central 's architecture). Note, D = 512 for our architecture means the central 's actor head has 512 • 13 3 =1,124,864 parameters. Contrast this to SYNC 's D × |A| × K parameters for a K mixture component. Even for the highest K in the mixture component study (Tab. 3), i.e., K = 13, this value is 86, 528 parameters. Such a large number of parameters makes learning with the central agent slow even after 1M episodes (this is already 10× more training episodes than used in [42] ).
Why MD-SPL instead of SPL? SPL was introduced in [2] for evaluating single-agent navigational agents, and is defined as follows:
EQUATION (5): Not extracted; please refer to original document.
where i denotes an index over episodes, N ep equals the number of test episodes, and S i is a binary indicator for success of episode i. Also x i is the length of the agent's path and l i is the shortest-path distance from agent's start location to the goal. Directly adopting SPL isn't pragmatic for two reasons:
(a) Coordinating actions at every timestep is critical to this multi-agent task. Therefore, the number of actions taken by agents instead of distance (say in meters) should be incorporated in the metric. (b) Shortest-path distance has been calculated for two agent systems for FurnLift [42] by finding the shortest path for each agent in a state graph. This can be done effectively for fairly independent agents. While each position of the agent corresponds to 4 states (if 4 rotations are possible), each position of the furniture object corresponds to # States = (#pos. for A 1 near obj) × (#pos. for A 2 near obj)
× (#rot. for obj) × (#rot. for A 1 ) × (#rot. for A 2 ),
This leads to 404,480 states for an agent-object-agent assembly. We found the shortest path algorithm to be intractable in a state graph of this magnitude. Hence we resort to the closest approximation of Manhattan distance from the object's start position to the goal's position. This is the shortest path, if there were no obstacles for navigation.
Minimal edits to resolve the above two problems lead us to using actions instead of distance, and leveraging Manhattan distance instead of shortest-path distance. This leads us to defining, as described in Section Sec. 6.2 of the main paper, the Manhattan distance based SPL (MDSPL) as the quantity
EQUATION (7): Not extracted; please refer to original document.
Defining additional metrics used for FurnLift. Jain et al . [42] use two metrics which they refer to as failed pickups (picked up, but not 'pickupable') and missed pickups ('pickupable' but not picked up). 'Pickupable' means when the object and agent configurations were valid for a PickUp action.
Plots for additional metrics. See Fig. 8, 9 , and 10 for plots of additional metric recorded during training for the FurnMove, Gridworld-FurnMove, and FurnLift tasks. Fig. 10 in particular shows how the failed pickups and missed pickups metrics described above are substantially improved when using our SYNC models.
Additional 3-agent experiments. In the main paper we present results when training SYNC , marginal, and central models to complete the 3-agent Gridworld-FurnMove task. We have also trained the same methods to complete the (visual) 3-agent FurnMove task. Rendering and simulating 3-agent interactions in AI2-THOR is computationally taxing. For this reason we trained our SYNC and central models for 300k episodes instead of the 500k episodes we used when training 2-agent models. As it showed no training progress, we also stopped the marginal model's training after 100k episodes. Training until 300k episodes took approximately four days using eight 12GB GPUs (∼ 768 GPU hours per model). After training, the SYNC , marginal, and central obtained a test-set success rate of 23.2 ± 2.6%, 0.0 ± 0.0%, and 12.4 ± 2.0% respectively. These results mirror those of the 3-agent Gridworld-FurnMove task from the main paper. Particularly, both the SYNC and central models train to reasonable success rates but the central model actually performs worse than the SYNC model. A discussion of our hypothesis for why this is the case can be found earlier in this section. In terms of our other illustrative metrics, our SYNC , marginal, and central respectively obtain MDSPL values of 0.029, 0.0, and 0.012, and Invalid prob values of 0.336, 0.854, and 0.132. Fig. 8 : Metrics recorded during training for the FurnMove task for various models. These plots add to the graph shown in Fig. 4a . Here solid lines indicate performance on the training set and dashed lines the performance on the validation set. For the Invalid prob and TVD metrics, only training set values are shown. For the TVD metric the black line (corresponding to the Marginal (w/o comm) model completely covers the green line corresponding to the Marginal model.
A.5 Qualitative Evaluation Details And A Statistical Analysis Of Learned Communication
Discussion of our qualitative video. We include a video of policy roll-outs in the supplementary material. This includes four clips, each corresponding to the rollout on a test scene of one of our models trained to complete the FurnMove task. Clip A. Marginal agents attempt to move the TV to the goal but get stuck in a narrow corridor as they struggle to successfully coordinate their actions. The episode is considered a failure as the agents do not reach the goal in the allotted 250 timesteps. A top-down summary of this trajectory is included in Fig. 11 . Clip B. Unlike the marginal agents from Clip A., in this clip two SYNC agents successfully coordinate actions and move the TV to the goal location in 186 steps. A top-down summary of this trajectory is included in Fig. 12 . Clip C. Here we show SYNC agents completing the Gridworld-FurnMove in a test scene (the same scene and initial starting positions as in Clip A and Clip B). The agents complete the task in 148 timesteps even after an initial search in the incorrect direction. weight of each agent into 128 evenly spaced bins corresponding to the 128 notes on a MIDI keyboard (0 corresponding to a frequency of ∼8.18 Hz and 127 to ∼12500 Hz). Next, we post-process the audio so that the communication from the agents is played on different channels (stereo) and has the Tech Bass tonal quality. As a result, the reader can experience what agent 1 hears (i.e., agent 2's reply weight) via the left earphone/speaker and what agent 2 hears (i.e., agent 1's reply weight) via the right speaker. In addition to the study in Sec. 6.4 and Sec. A.5, we notice a higher pitch/frequency for the agent which is passing. We also notice lower pitches for MoveWithObject and MoveObject actions.
Joint policy summaries. These provide a way to visualize the effective joint distribution that each method captures. For each episode in the test set, we log each multi-action attempted by a method. We average over steps in the episode to obtain a matrix (which sums to one). Afterwards, we average these matrices (one for each episode) to create a joint policy summary of the method for the entire test set. This two-staged averaging prevents the snapshot from being skewed towards actions enacted in longer (failed or challenging) episodes. In the main paper, we included snapshots for FurnMove in Fig. 5 . In Fig. 13 we include additional visualizations for all methods including (Marginal w/o comm model) for FurnMove and Gridworld-FurnMove. Fig. 10 : Metrics recorded during training for the FurnLift task for various models. These plots add to the graph shown in Fig. 4c . Notice that we have included plots corresponding to the failed pickups (picked up, but not 'pickupable') and missed pickups ('pickupable' but not picked up) metrics described in Sec. A.4. Solid lines indicate performance on the training set and dashed lines the performance on the validation set. For the Invalid prob and TVD metrics, only training set values are shown. For the TVD metric the black line (corresponding to the Marginal (w/o comm) model completely covers the green line corresponding to the Marginal model.
Communication Analysis.
As shown in Fig. 5d and discussed in Sec. 6.4, there is very strong qualitative evidence suggesting that our agents use their talk and reply communication channels to explicitly relay their intentions and coordinate their actions. We now produce a statistical, quantitative, evaluation of this phenomenon by fitting multiple logistic regression models where we attempt to predict, from the agents communications, certain aspects of their environment as well as their future actions. In particular, we run 1000 episodes on our test set using our mixture model in the visual testbed. This produces a dataset of 159,380 observations where each observation records, for a single step by both agents at time t:
(a) The two weights p 1 talk,t , p 2 talk,t where p i talk,t is the weight agent A i assigns to the first symbol in the "talk" vocabulary. (b) The two weights p 1 reply,t , p 2 reply,t where p i reply,t is the weight agent A i assigns to the first symbol in the "reply" vocabulary. (c) The two values tv i t ∈ {0, 1} where tv i t equals 1 if and only if agent A i sees the TV at timestep t (before taking its action). (d) The two values WillPass i t ∈ {0, 1} where WillPass i t equals 1 if and only if agent i ends up choosing to take the Pass action at time t (i.e., after finishing communication). (e) The two values WillMWO i t ∈ {0, 1} where WillMWO i t equals 1 if and only if agent i ends up choosing to take some MoveWithObject action at time t.
In the following we will drop the subscript t and consider the above quantities as random samples drawn from the distribution of possible steps taken by our agents in randomly initialized trajectories. As A 1 and A 2 share almost all of their parameters they are, essentially, interchangeable. Because of this our following analysis will be solely taking the perspective of agent A 1 , similar results hold for A 2 . We consider fitting the three models:
+ 2 i=1 β i talk, MWO • p i talk + 2 i=1 β i reply, MWO • p i reply ,
where σ is the usual logistic function. Here Eq. (8) attempts to determine the relationship between what A 1 communicates and whether or not A 1 is currently Fig. 11 : Clip A trajectory summary. The marginal agents quickly get stuck in a narrow area between a sofa and the wall and fail to make progress.
seeing the TV, Eq. (9) probes whether or not any communication symbol is associated with A 1 choosing to take a Pass action, and finally Eq. (10) considers whether or not A 1 will choose to take a MoveWithObject action. We fit each of the above models using the glm function in the R programming language [73] . Moreover, we compute confidence intervals for our coefficient values using a robust bootstrap procedure. Fitted parameter values can be found in Tab. 9. From Tab. 9 we draw several conclusions. First, in our dataset, there is a somewhat complex association between agent A 1 seeing the TV and the communication symbols it sends. In particular, for a fixed reply weight p 1 reply < 0.821, a larger value of p 1 talk is associated with higher odds of the TV being visible to A 1 but if p 1 reply > 0.821 then larger values of p 1 talk are associated with smaller odds of the TV being visible. When considering whether or not A 1 will pass, the table shows that this decision is strongly associated with the value of p 2 reply
Episode Start
Progress after 62 steps Progress after 124 steps Progress after 186 steps (success) Fig. 12 : Clip B trajectory summary. The SYNC agents successfully navigate the TV to the goal location without getting stuck in the narrow corridor. where, given fixed values for the other talk and reply weights, p 2 reply being larger by a unit of 0.1 is associated with 2.7× larger odds of A 1 taking the pass action. This suggests the interpretation of a large value of p 2 reply as A 2 communicating that it wishes A 1 to pass so that A 1 may perform a single-agent navigation action to reposition itself. Finally, when considering the fitted values corresponding to Eq. (10) we see that while the talk symbols communicated by the agents are weakly related with whether or not A 1 takes a MoveWithObject action, the reply symbols are associated with coefficients with an order of magnitude larger values. In particular, assuming all other communication values are fixed, a smaller value of either p 1 reply or p 2 reply is associated with substantially larger odds of A 1 choosing a MoveWithObject action. This suggests interpreting an especially small value of p i reply as agent A i indicating its readiness to move the object.
For FurnMove, each location of the lifted furniture corresponds to 404, 480 states, making shortest path computation intractable (more details in Sec. A.4 of the supplement).